A project’s dependency on resources’ (average) utilization time (UT) and quality expectations expressed as a quality factor (QF) doesn’t come as a surprise, as hopefully one is acquainted with project’s triangle which reflects the dependency between scope, cost and time in respect to quality. Even if this dependency is intuitive, it’s difficult to express it in numbers and study the way it affects the project. That was the purpose of the model built previously.
A Software Engineer and data professional's blog on SQL, data, databases, data architectures, data management, programming, Software Engineering, Project Management, ERP implementation and other IT related topics.
Pages
- 🏠Home
- 🗃️Definitions
- 🔢SQL Server
- 🎞️SQL Server: VoD
- 🏭Fabric
- 🎞️Fabric: VoD
- ⚡Power BI
- 🎞️Power BI: VoD
- 📚Data
- 📚Engineering
- 📚Management
- 📚SQL Server
- 🎞️D365: VoD
- 📚Systems Thinking
- ✂...Quotes
- 🧾D365: GL
- 💸D365: AP
- 💰D365: AR
- 🏠D365: FA
- 👥D365: HR
- ⛓️D365: SCM
- 🔤Acronyms
- 🪢Experts
- 🗃️Quotes
- 🔠Dataviz & BI
- 🔠D365
- 🔠Fabric
- 🔠Engineering
- 🔠Management
- 🔡Glossary
- 🌐Resources
- 🏺Dataviz
- 🗺️Social
- 📅Events
- ℹ️ About
20 May 2020
💼Project Management: Project Planning (Part V: Some Thoughts on Planning II)
A project’s dependency on resources’ (average) utilization time (UT) and quality expectations expressed as a quality factor (QF) doesn’t come as a surprise, as hopefully one is acquainted with project’s triangle which reflects the dependency between scope, cost and time in respect to quality. Even if this dependency is intuitive, it’s difficult to express it in numbers and study the way it affects the project. That was the purpose of the model built previously.
💎🏭SQL Reloaded: Query Patterns in SQL Server (Part V: Matrix Representation)
I have made mainly two changes to the old example - I defined the start, end and step to be numeric, while considering also an index into the output table. Here’s the modified function:
-- SQL Server 2005+: Numeric Interval CREATE FUNCTION dbo.fGetNInterval( @Start numeric(18,2) , @End numeric(18,2) , @Step numeric(18,2)) RETURNS @Interval TABLE( Ind int PRIMARY KEY , Val numeric(18,2)) AS BEGIN DECLARE @Sign smallint SET @Sign = IsNull(NullIf(Sign(@End-@Start), 0), 1); WITH CTE(Ind, Val) AS( SELECT Cast(1 as int) Ind , Cast(COALESCE(@Start, @End, 0) as numeric(18,2)) Val UNION ALL SELECT cast(Ind+1 as int) Ind , Cast(CTE.Val + @Step * @Sign as numeric(18,2)) Val FROM CTE WHERE (@End-CTE.Val)*@Sign-@Step>=0 ) INSERT @Interval SELECT Ind , Val FROM CTE OPTION(MAXRECURSION 0) RETURN END
--descending sequence SELECT UT.Val, 100/UT.Val FROM dbo.fGetNInterval(100, 25, 5) UT -- ascending sequence SELECT * FROM dbo.fGetNInterval(1, 2, 0.25)
-- matrix representation DECLARE @EE as numeric(18,2) = 100 SELECT UT.Val [UT %] , Max(CASE WHEN QF.Ind = 1 THEN Cast(QF.Val*@EE*100/UT.Val as numeric(18,1)) END) [80%] , Max(CASE WHEN QF.Ind = 2 THEN Cast(QF.Val*@EE*100/UT.Val as numeric(18,1)) END) [85%] , Max(CASE WHEN QF.Ind = 3 THEN Cast(QF.Val*@EE*100/UT.Val as numeric(18,1)) END) [90%] , Max(CASE WHEN QF.Ind = 4 THEN Cast(QF.Val*@EE*100/UT.Val as numeric(18,1)) END) [95%] , Max(CASE WHEN QF.Ind = 5 THEN Cast(QF.Val*@EE*100/UT.Val as numeric(18,1)) END) [100%] FROM dbo.fGetNInterval(100, 25, 5) UT , dbo.fGetNInterval(1, 2, 0.25) QF GROUP BY UT.Ind , UT.Val
Here’s the output:
Note:
The indexes were used to display the values into the needed format. The table-valued function could be created also without the respective indexes, however it would have complicated the query.
19 May 2020
💼Project Management: Project Planning (Part IV: Some Thoughts on Planning I)
One of the issues in Project Management (PM) planning is that the planner idealizes a resource and activities performed by it much like a machine. Unlike machines whose uptime can approach 100%, a human resource can work at most 90% of the available time (aka utilization time), the remaining 10% being typically associated with interruptions – internal emails and meetings, casual communications, pauses, etc. For resources split between projects or operations the utilization time can be at most 70%, however a realistic value is in general between 40% and 60% on average. What does it mean this for a project?
📦Data Migrations (DM): In-house Built Solutions (Part I - An Introduction)
Data Migrations Series |
17 May 2020
💎🏭SQL Reloaded: Query Patterns in SQL Server (Part IV: Window Functions)
For a long time aggregate functions were the only tool for statistical purposes available for raw SQL scripting. Their limitations become more evident with the introduction of window functions, which allow to apply the aggregates over a defined partition. Besides aggregate window functions, the use of ranking, respectively value window functions, opened the door to a new set of techniques. Here are a few examples based on the tables defined in a previous post.
Ranking windows functions allow ranking a record within the whole dataset or a partition by providing a sorting key:
-- Ranking window functions (RANK, DENSE_RANK, ROW_NUMBER) SELECT A.CourseId , C.CourseName , A.StudentId , S.StudentName , A.StartDate , A.EndDate , RANK() OVER (ORDER BY A.Mark) [Rank over whole set] , DENSE_RANK() OVER (ORDER BY A.Mark) [Dense Rank over whole set] , ROW_NUMBER() OVER (ORDER BY A.Mark) [Row Number over whole set] --, RANK() OVER (PARTITION BY A.CourseId ORDER BY A.StartDate) [Rank over Course] --, RANK() OVER (PARTITION BY A.StudentId ORDER BY A.StartDate) [Rank over Student] --, DENSE_RANK() OVER (PARTITION BY A.CourseId ORDER BY A.StartDate) [Dense Rank over Course] --, DENSE_RANK() OVER (PARTITION BY A.StudentId ORDER BY A.StartDate) [Dense Rank over Student] --, ROW_NUMBER() OVER (PARTITION BY A.CourseId ORDER BY A.StartDate) [Dense Rank over Course] --, ROW_NUMBER() OVER (PARTITION BY A.StudentId ORDER BY A.StartDate) [Dense Rank over Student] FROM dbo.T_Allocations A JOIN dbo.T_Courses C ON A.CourseId = C.CourseId JOIN dbo.T_Students S ON A.StudentId = S.StudentId ORDER BY C.CourseName , S.StudentName
Being able to rank records allows to easier select the last, respectively the first records from a dataset or a given partition:
-- first course SELECT * , A.StartDate FROM dbo.T_Students S LEFT JOIN ( SELECT A.CourseId , A.StudentId , A.StartDate , RANK() OVER (PARTITION BY A.StudentId ORDER BY A.StartDate) Ranking FROM dbo.T_Allocations A ) A ON S.StudentId = A.StudentId AND A.Ranking = 1 ORDER BY S.StudentName
The aggregate window functions function similarly as their simple counterparts, except that they are valid over a partition. If the partition is left out, the functions apply over the whole data set.
-- aggregate window functions SELECT A.CourseId , C.CourseName , A.StudentId , S.StudentName , A.StartDate , A.EndDate , SUM(A.Mark) OVER (PARTITION BY A.StudentId) [Sum] , MAX(A.Mark) OVER (PARTITION BY A.StudentId) [Max] , MIN(A.Mark) OVER (PARTITION BY A.StudentId) [Min] , AVG(A.Mark) OVER (PARTITION BY A.StudentId) [Avg] , COUNT(A.Mark) OVER (PARTITION BY A.StudentId) [Count] FROM dbo.T_Allocations A JOIN dbo.T_Courses C ON A.CourseId = C.CourseId JOIN dbo.T_Students S ON A.StudentId = S.StudentId ORDER BY C.CourseName , S.StudentName
When the ordering attributes are specified, running averages, respectively sums derive:
-- Running averages/sums and ranking via Sum and ORDER BY SELECT A.StudentId , A.CourseId , A.StartDate , A.EndDate , A.Mark , AVG(Cast(Mark as decimal(4,2))) OVER (PARTITION BY A.StudentId ORDER BY A.StartDate, A.AllocationId) AvgGrade , SUM(Mark) OVER (PARTITION BY A.StudentId ORDER BY A.StartDate, A.AllocationId) SumGrade , RANK() OVER (PARTITION BY A.StudentId ORDER BY A.StartDate, A.AllocationId) Ranking FROM dbo.T_Allocations A ORDER BY A.StudentId , A.StartDate
-- Running averages/sums and ranking via common table expressions WITH CTE AS ( SELECT A.StudentId , A.CourseId , A.StartDate , A.EndDate , A.Mark , RANK() OVER (PARTITION BY A.StudentId ORDER BY A.StartDate, A.AllocationId) Ranking FROM dbo.T_Allocations A ), DAT AS ( SELECT A.StudentId , A.CourseId , A.StartDate , A.EndDate , A.Mark , A.Ranking , A.Mark SumMark FROM CTE A WHERE A.Ranking = 1 UNION ALL SELECT CTE.StudentId , CTE.CourseId , CTE.StartDate , CTE.EndDate , CTE.Mark , CTE.Ranking , CTE.Mark + DAT.Mark SumMark FROM CTE JOIN DAT ON CTE.StudentID = DAT.StudentId AND CTE.Ranking-1 = DAT.Ranking ) SELECT DAT.StudentId , DAT.CourseId , DAT.StartDate , DAT.EndDate , DAT.Mark , cast(DAT.SumMark/DAT.Ranking as decimal(4,2)) AvgMark , DAT.SumMark , DAT.Ranking FROM DAT ORDER BY DAT.StudentId , DAT.StartDate
💎🏭SQL Reloaded: Query Patterns in SQL Server (Part III: Aggregate Functions)
-- aggreates over the whole table SELECT COUNT(*) NoRecords , MIN(StartDate) StartDate , MAX(EndDate) EndDate , DateDiff(d, MIN(StartDate), MAX(EndDate)) [DiffDays] FROM dbo.T_Allocations A -- aggregates based on several several attributes SELECT A.CourseId , A.StudentId , COUNT(*) NoRecords , MIN(StartDate) StartDate , MAX(EndDate) EndDate , DateDiff(d, MIN(StartDate), MAX(EndDate)) [DiffDays] FROM dbo.T_Allocations A GROUP BY A.CourseId , A.StudentId
-- correlated subquery within a SELECT SELECT * , (SELECT COUNT(*) NoRecords FROM dbo.T_Allocations A WHERE C.CourseId = A.CourseId GROUP BY A.CourseId) NoRecords FROM dbo.T_Courses C -- CROSS APPLY (correlated subquery) SELECT * , A.NoRecords , A.StartDate FROM dbo.T_Courses C CROSS APPLY ( SELECT A.CourseId , COUNT(*) NoRecords , MIN(StartDate) StartDate FROM dbo.T_Allocations A WHERE C.CourseId = A.CourseId GROUP BY A.CourseId ) A ORDER BY C.CourseName -- OUTER APPLY (correlated subquery) SELECT * , A.NoRecords , A.StartDate FROM dbo.T_Courses C OUTER APPLY ( SELECT A.CourseId , COUNT(*) NoRecords , MIN(StartDate) StartDate FROM dbo.T_Allocations A WHERE C.CourseId = A.CourseId GROUP BY A.CourseId ) A ORDER BY C.CourseName
-- identifying duplicates via an aggregate SELECT A.* FROM dbo.T_Allocations A JOIN ( SELECT A.CourseId , A.StudentId , COUNT(*) NoRecords FROM dbo.T_Allocations A GROUP BY A.CourseId , A.StudentId HAVING COUNT(*)>1 ) DUP ON A.CourseId = DUP.CourseId AND A.StudentId = DUP.Studentid ORDER BY A.CourseId , A.StudentId
-- removing the duplicates except the last record DELETE dbo.T_Allocations FROM ( SELECT A.StudentId , A.CourseId , A.StartDate , A.EndDate , Max(A.AllocationId) AllocationId FROM dbo.T_Allocations A GROUP BY A.StudentId , A.CourseId , A.StartDate , A.EndDate HAVING count(*)>1 ) A WHERE dbo.T_Allocations.CourseId = A.CourseId AND dbo.T_Allocations.StudentId = A.StudentId AND dbo.T_Allocations.StartDate = A.StartDate AND dbo.T_Allocations.EndDate = A.EndDate AND dbo.T_Allocations.AllocationId <> A.AllocationId
-- Matrix display via aggregates SELECT S.StudentId , S.StudentName , CASE WHEN A.Course1 = 1 THEN 'x' ELSE '-' END Course1 , CASE WHEN A.Course2 = 1 THEN 'x' ELSE '-' END Course2 , CASE WHEN A.Course3 = 1 THEN 'x' ELSE '-' END Course3 , CASE WHEN A.Course4 = 1 THEN 'x' ELSE '-' END Course4 , CASE WHEN A.Course5 = 1 THEN 'x' ELSE '-' END Course5 FROM dbo.T_Students S LEFT JOIN ( SELECT A.StudentId , Max(CASE WHEN A.CourseId = 1 THEN 1 ELSE 0 END) Course1 , Max(CASE WHEN A.CourseId = 2 THEN 1 ELSE 0 END) Course2 , Max(CASE WHEN A.CourseId = 3 THEN 1 ELSE 0 END) Course3 , Max(CASE WHEN A.CourseId = 4 THEN 1 ELSE 0 END) Course4 , Max(CASE WHEN A.CourseId = 5 THEN 1 ELSE 0 END) Course5 FROM dbo.T_Allocations A GROUP BY A.StudentId ) A ON S.StudentId = A.StudentId ORDER BY S.StudentName
16 May 2020
💎🏭SQL Reloaded: Query Patterns in SQL Server (Part II: Joins and Subqueries)
The basis for being able to manipulate data via SQL scripting is a good knowledge of using joins and subqueries as seems fit for the purpose and data models. The following scripts are based on the tables created in a previous post.
-- FULL JOIN SELECT * FROM dbo.T_Courses C JOIN dbo.T_Students S ON C.CourseId = S.CourseId ORDER BY C.CourseName , S.StudentName -- FULL JOIN (deprecated) SELECT * FROM dbo.T_Courses C , dbo.T_Students S WHERE C.CourseId = S.CourseId ORDER BY C.CourseName , S.StudentName --LEFT JOIN SELECT * FROM dbo.T_Courses C LEFT JOIN dbo.T_Students S ON C.CourseId = S.CourseId WHERE S.CourseId IS NULL ORDER BY C.CourseName , S.StudentName -- RIGHT JOIN SELECT * FROM dbo.T_Courses C RIGHT JOIN dbo.T_Students S ON C.CourseId = S.CourseId WHERE C.CourseId IS NULL ORDER BY C.CourseName , S.StudentName -- FULL OUTER JOIN SELECT * FROM dbo.T_Courses C FULL OUTER JOIN dbo.T_Students S ON C.CourseId = S.CourseId --WHERE C.CourseId IS NULL --WHERE S.CourseId IS NULL --WHERE (C.CourseId IS NULL OR S.StudentId IS NULL) --WHERE (C.CourseId IS NULL AND S.StudentId IS NULL) ORDER BY C.CourseName , S.StudentName
The IN, NOT IN, EXISTS and NOT EXISTS allow using correlated queries, their use being indicated when there are no actual data needed from the tables involved in the correlated queries:
-- EXISTS (correlated subquery) SELECT * FROM dbo.T_Courses C WHERE EXISTS (SELECT StudentId FROM dbo.T_Students S WHERE C.CourseId = S.CourseId) ORDER BY C.CourseName -- NOT EXISTS (correlated subquery) SELECT * FROM dbo.T_Courses C WHERE EXISTS (SELECT StudentId FROM dbo.T_Students S WHERE C.CourseId = S.CourseId) ORDER BY C.CourseName -- IN (subquery) SELECT * FROM dbo.T_Courses C WHERE CourseId IN (SELECT CourseId FROM dbo.T_Students S) ORDER BY C.CourseName
Joining multiples tables is done using the same principles as above:
-- joins with more tables SELECT A.CourseId , C.CourseName , A.StudentId , S.StudentName , A.StartDate , A.EndDate FROM dbo.T_Allocations A JOIN dbo.T_Courses C ON A.CourseId = C.CourseId JOIN dbo.T_Students S ON A.StudentId = S.StudentId ORDER BY C.CourseName , S.StudentName
One can obtain the same result via correlated subqueries (a technique often met between Oracle developers). From readability reasons I avoid writing such queries, unless there’s a special purpose to do so.
-- correlated subquery for individual values SELECT A.CourseId , (SELECT C.CourseName FROM dbo.T_Courses C WHERE A.CourseId = C.CourseId) CourseName , A.StudentId , (SELECT S.StudentName FROM dbo.T_Students S WHERE A.StudentId = S.StudentId) StudentName , A.StartDate , A.EndDate FROM dbo.T_Allocations A ORDER BY CourseName , StudentName
When displaying values within a SELECT via a correlated subqueries, some developers feel the need to use MAX or MIN functions to make sure only one value will be returned. For data analysis it may be acceptable, however if the data model imposes it, then a redesign of the solution is more likely necessary.
-- correlated subquery for individual values SELECT A.CourseId , (SELECT Max(C.CourseName) FROM dbo.T_Courses C WHERE A.CourseId = C.CourseId) CourseName , A.StudentId , (SELECT Max(S.StudentName) FROM dbo.T_Students S WHERE A.StudentId = S.StudentId) StudentName , A.StartDate , A.EndDate FROM dbo.T_Allocations A ORDER BY CourseName , StudentName
Another technique not recommended is displaying one or more attributes from the same table with the same conditions via individual correlated queries. The use of aggregate functions is more appropriate however with numerical or date values.
Notes:
The queries work also in SQL databases in Microsoft Fabric.
Happy coding!
Previous Post <<||>> Next Post
About Me
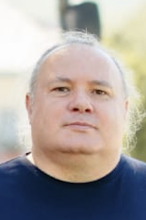
- Adrian
- Koeln, NRW, Germany
- IT Professional with more than 25 years experience in IT in the area of full life-cycle of Web/Desktop/Database Applications Development, Software Engineering, Consultancy, Data Management, Data Quality, Data Migrations, Reporting, ERP implementations & support, Team/Project/IT Management, etc.