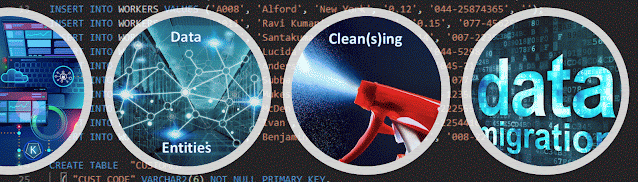 |
Data Migrations Series |
IntroductionBasically,
Data Migration is the movement of data from one IS (Information System), the legacy system, to a new IS, the target system, supposed to replace entirely or partially the legacy system. In the best scenario there are no differences between the two IS or the differences are minimal, negligible. In the worst scenario, there are multiple legacy systems used as source, and even multiple target systems, with important differences between them, differences that can even be translated in incompatibilities at multiple levels. Such architectures can span geographies, departments, organizations or industries; can involve a multitude of vendors, generations of systems, network types, different regulations, etc. In many Data Migrations the overall picture can be really complex, though for the sake of simplicity it’s enough to focus on the simplest scenario in which there is a single source and a single target system, with some differences between them. Abstraction can be made also of the fact that many migrations are parts of bigger projects, for example ERP implementations or any other type of applications migrations.
Data Migration is quite a complex topic, for many appearing like a black box in which data come in and data come out. That’s valid for the typical user as well for the IT professionals who haven’t been involved in Data Migration projects. There are many books on topics that are tangent to Data Migration – Data Management, Data Quality, Data Integration or Data Warehousing. Excepting some presentations available on the Web, a few methodologies exposed by important companies, one or two books, and a few blogs, there isn’t much material available on Data Migration. The “trend” is also a reflection of the low importance given to Data Migration as subject, even if many professionals working in the field warn about the considerable impact a Data Migration can have on a project in particular, and on business in general.
Approaching a topic like Data Migration can be, upon case, a complex task, however with a little intuition and some guidance its complexity falls apart. Often, when exploring such a topic, of help can be the 5W1H technique or its extended forms. The technique resumes to searching for answers to the “what”, “where”, “why”, “how”, “when”, “who” and “with what” questions. In case of Data Migration the questions are formulated as: what (data) to migrate, where to migrate, why to migrate, how to migrate, when to migrate, who migrates and with what to migrate?
Why to migrate?A Data Migration occurs as follow up of a need – an old system exists in place and can’t cope anymore with business’ growth, a company made an acquisition and the systems need to be consolidated, or the organization decided to change its infrastructure, the processes, the business model in order address nowadays business requirements like flexibility, availability, manageability, automation, cost cuts, etc. In other words a Data Migration occurs as a need for change, and it can be itself a change in what concerns technical infrastructure, process, procedures, data flow, ways of doing business. A migration has quite an impact on the business, so here is an entitled question: does it really makes sense to migrate? Why not start from 0 with the new system?!
The migration can be a 0 point for an organization, though unless a company is starting anew, there are some data laying there in the old system(s) that need to be further available - for example open Purchase Orders that need to be fulfilled, Invoices that need to be paid, a catalog with all the Products and the available stock, information about Customers, what they bought, what they browsed or what they want to buy for Christmas, etc. At least some of the data need to be made available in one form and another also within the new architecture, if not the new system.
The availability of old data can be solved by keeping the old system(s) in place, functional, even if the system won’t be fed with new data, or maybe it will. Keeping a system alive involves additional costs for maintaining the infrastructure – software and hardware licenses, consultants, administrators and other people responsible for the optimal work of such a system. This can become with time quite an unnecessary burden. It can be an acceptable choice for some organizations, but unlikely as best/good practice. And even if the system is kept, more likely there will be data that need to be available also in the new system. Can be discussed also about integration of the two systems, but again, does it make sense? The bottom line is that in multiple scenarios a Data Migration can prove to be the optimal solution for an organization.
What data to migrate?Even if it looks like a silly question, it can be one of most complex questions to answer. In theory is needed to migrate all the data, but are really needed all the data? Typically in a database can be found historical data not used anymore by the business, obsolete data marked or not for deletion, garbage data entered by mistake or remained after incomplete deletions, all these having low or no value for the business. Hopefully there are also “good data”, quintessential for the business. Somebody would say “what a hack, why do we need to philosophize so much, let’s migrate all the data!”. The decision can be understandable, though what if the percentage of “good data” is quite small in comparison with the total volume of data which can measure a few terabytes?! Sure, nowadays data centers can handle without problems terabytes of data, though there are some factors to be considered – it can be quite a challenge to migrate so many data, the volume of data affects also the performance of databases in particular, and IS in general, and a more natural reason – why store something that has minimal value for you?!
It makes sense to migrate only the data that have value for an organization, but what data are needed then? Normally this starts by understanding what entities the business deals with and which are the attributes that characterizes them. Many of the entities can be met in organization’s daily activity, and maybe are already defined in organization’s glossary or Data Dictionary, so a review of the available inventory might do. If not, more effort needs to be spent for this purpose;
activities specific to Data Discovery, Data Categorization, Data Definition or Data Profiling tasks can help after case to fill the understanding gaps. Except categorization the others are not all necessary, same as the analysis can be deep enough to serve the purpose.
A first categorization was made above when data were considered as valuable, not valuable or in between. A second categorization can be made based on data’s usage: obsolete (not used anymore or marked for deletion), new (not used and recently entered), historical (data used in the past) and actual (data in use). A third categorization can be made on the status of the entities they represent, status that can be associated to the phase of the process the entity represent (e.g. active, inactive, open, invoices, closed, blocked, etc.). There can be considered other meaningful categorizations as long they prove to be important in identifying the useful data.
An important categorization in migrations, in particular, and Data Management, in general, is to split data in master data, transaction data and setup data. Master data are data are data that change only seldom and have a long life (until become obsolete), are referenced through all the system, and are vital to an organization through their meaning (e.g. Customers, Suppliers, Products, Assets, Employees, Accounts, etc.). Transaction data in exchange are data that change often and have a relatively short life, typically are referenced by other transactions and can be associated with documents or movements through the system (e.g. Purchase Orders, Sales Orders, Invoices, Receipts, Assets Movements, etc.). Setup data are data used to configure a system (e.g. Transaction Types, Document Types, Roles, Permissions, etc.). This categorization deserves the full attention, because each of the three elements needs a different handling approach in migration or Data Management.
Based on the identified categories can be considered some rough migration rules in deciding what data (actually records) to migrate, for example:
- master data, unless they become obsolete, and open transactions are often considered to be migrated entirely;
- historical transaction data spanning a few years back can be migrated in case they are needed in the process;
- master data referenced by transaction data migrated need to be migrated too
- setup data are entered manually
- historical data are archived. There can be also exceptions from the rules, so such possible scenarios need to be considered too.
Each entity is defined by multiple attributes (also called properties, dimensions). They need to go through a similar “categorization” process. In deciding what attributes to migrate is important to consider especially their role in defining the entity. Some of them define uniquely an entity (e.g. Customer Number, Product Number, Serial Number), physical characteristics of the entity (e.g. color, weight, height), categorize the entity (e.g. Category, Type) or its status (e.g. Active, Blocked, Invoiced), imply various events (e.g. Creation Date, Delivery Date, Invoice Date), and so on. It looks like another type of categorization, and it is, though it’s more difficult to create some rough rules based on it, because in the end the business dictates which Attributes are needed. In fact, most of the Attributes used (with distinct not null values) in the legacy system are more likely needed also in the new system, unless the process changed considerably, or the business is supposed to change also its model.
Where to migrate the data?When the Data Migration subject is brought on the table, a decision was already made about the target system. So the “where” question is partially answered, however it addresses only the peak of the iceberg. It shows that an iceberg lies there, in front of us, though under the deep of the waters there is something more, lot of questions and issues that need to be addressed. Like the source, the target needs to be further detailed in entities and their attributes; the targeted processes and procedures need to be considered together with the constraints imposed by the new system. It’s actually needed to identify the data requirements for the new systems and corroborate them with the requirements of the old system. Mapping the entities and attributes available in the two systems, process known as Data Mapping, can offer a good overview of what lays ahead, what similarities and gaps exist. There will be attributes that are available in the legacy but not in the target system, and therefore the target system needs to be extended or the data associated with the respective attributes can be left out. From the opposed perspective, there can be mandatory attributes in the target system which are not available in the organization, and therefore the associated data must be collected and/or made available for the migration. There can be cases when the data are not available in the legacy system but distributed in various other external or internal sources, so there can be an option to migrate or integrate the respective data, extend the processes to accommodate such scenarios, etc.
Only when the mapping of data is ready and the various related questions addressed, the “where” question is fully answered. Given the continuous changes done to the target system that may still happen a few days before Go Live, Data Mapping can remain a hot topic until then.
With what to migrate?This question addresses the mix of tools used to migrate the data, and by extension the whole architecture developed for this purpose. As many experts point out, there is no general solution for such an approach because each migration is challenged by different requirements and architectures. ETL (Extract, Transform, Load) and Data Integration tools were mainly designed for this kind of purposes – moving data between data sources – therefore more likely the whole Data Migration architecture will be built around such a tool. In addition is needed to be addressed topics like assessment and reporting of Data Quality, Data Cleaning, Data Enrichment, Data Backup or Data Security. They will technically ensure that the data are migrated within intended level of quality and security.
For each of these topics are available one or more tools on the market. The challenge is to find the right mixture for the overall architecture, to make them work together in an efficient and effective manner. One of the problems such tools have is that they look to the Data Migration or similar problems from their own perspective, making them hard to integrate with other tools. Given the increasing need for Data Migration, more likely exist there tools that cover most of its requirements, each with its own advantages and disadvantages. Starting with a new tool can prove to be quite challenge in itself. Many recommend following a methodology and using tools that already proved their capabilities in other projects. That’s a good approach, though need to be considered also costs, available resources, effort to build the infrastructure, the learning curve, etc. For some migrations MS Excel or Access will do, for others a more complex framework is needed. Keep in mind that there is no perfect architecture, just the architecture that will drive you to achieve your targets.
How to migrate the data?“How” refers mainly to the migration approach, steps, methodologies, processes and procedures used to migrate the data. Secondly, and not less important, it refers to how the mix of tools is used for migration – in other words the implementation. Despite the huge variety of tools and means of achieving the target, there can be depicted some generalities for each of these topics.
Migration approach refers to the overall strategy considered for a migration – typically on whether the data are migrated all together, the new system becoming functional and replacing the legacy system (the big-bang migration), or the data are migrated in phases, the legacy and target systems functioning in parallel for a certain amount of time (the phased-out migration). Can be met other variations of migration approaches, under various denominations. It’s important to know the advantages and disadvantages of both or all approaches, especially in what concerns their application in your organization.
“Steps” is just a misnomer for the actual Project Plan in which are considered the different phases and activities of such a project. In a general Data Migration project, can be discussed about Data Discovery, Data Definition, Data Collection, Data Consolidation, Data Mapping, Data Conversion, Data Transformation, Data Quality Assessment, Data Cleaning, Data Storage, etc. Some of these steps can be considered as standalone processes, sometimes being already part of the processes’ landscape existing in an organization. Other steps are just simple activities. Both types of steps share some important characteristics – they can be highly iterative and complex, are owned by the business, the IT functioning as facilitator, each of them depends on the input from other steps, and require continuous feedback, etc.
A Data Migration is (should be) managed as any other IT project, and therefore can be discussed about project-specific methodologies like PMBOK, Prince2 or PRISM. Many of the before mentioned steps come with their luggage of methodologies too. In addition, considering that IT functions as a service, could be considered service-specific methodologies like ITIL, ISO/IEC, Six Sigma, etc.
The actual implementation of all these depends entirely on the project’s scope, the knowledge of all those involved, the constraints met and the resources available for such a project. Many of the IT-specific problems and situations are specific across all IT projects.
Who will migrate the data?There is no Data Migration project that can be done without the business, the de facto owner of such a project and its output. There is lot of input needed from the business, its continuous involvement through the various stages is necessary for the whole duration. Unless the Data Migration resumes to a rudimentary tool like Excel and can be handled without too much expertise, a Data Migration needs technical resources that can elicit the requirements, translate them in technical requirements, built the infrastructure and maybe migrate the data. It entirely depends on the overall architecture and methodology what people are involved. In the best case scenario the migration will resume to one person pushing a button and the data flow as magic from source to the target system. In reality, multiple people will have to take care of migration, pushing some magic buttons in a chain of parallel and even redundant steps, monitoring and validating the process. Data owners, data stewards, data custodians, data architects, database administrators, migration and quality assurance specialists, developers, consultants and many other people can be involved, each of them playing their role.
When to migrate the data?Intuitively, data are or should be migrated when the target system is ready to receive the new data, thus when the development was finished, the system tested, and all the preparation for Data Migration were made. The statement is valid for any type of migration. How such a date or dates are calculated when a project starts is in itself kind of science or just a matter of needs. There are projects in which the dates for each milestone or phase are calculated back from a desired Go Live date, or projects in which the Go Live is calculated incrementally based on the steps to be performed. For dates’ calculation can be used also benchmarking from the field. The bottom line is that the data must be migrated on time for the Go Live and with a minimum disruption for the business.
ConclusionWhether standalone or as subproject of another project, a Data Migration can be or become quite a complex thematic that, through its outcomes, affects the business considerably. In the above paragraphs were considered some of the important aspects of such a project, the focus being more on figuring out what a migration implies rather than a detailed exploration. It’s also a mental exercise and an invitation into the thematic.