![]() |
Data Visualization Series |
A Software Engineer and data professional's blog on SQL, data, databases, data architectures, data management, programming, Software Engineering, Project Management, ERP implementation and other IT related topics.
Pages
- 🏠Home
- 🗃️Posts
- 🗃️Definitions
- 🔢SQL Server
- 🎞️SQL Server: VoD
- 🏭Fabric
- 🎞️Fabric: VoD
- ⚡Power BI
- 🎞️Power BI: VoD
- 📚Data
- 📚Engineering
- 📚Management
- 📚SQL Server
- 🎞️D365: VoD
- 📚Systems Thinking
- ✂...Quotes
- 🧾D365: GL
- 💸D365: AP
- 💰D365: AR
- 🏠D365: FA
- 👥D365: HR
- ⛓️D365: SCM
- 🔤Acronyms
- 🪢Experts
- 🗃️Quotes
- 🔠Dataviz & BI
- 🔠D365
- 🔠Fabric
- 🔠Engineering
- 🔠Management
- 🔡Glossary
- 🌐Resources
- 🏺Dataviz
- 🗺️Social
- 📅Events
- ℹ️ About
14 December 2024
🧭💹Business Intelligence: Perspectives (Part XXI: Data Visualization Revised)
13 December 2024
🧭💹Business Intelligence: Perspectives (Part XX: From BI to AI)
![]() |
Business Intelligence Series |
No matter how good data visualizations, reports or other forms of BI artifacts are, they only serve a set of purposes for a limited amount of time, limited audience or any other factors that influence their lifespan. Sooner or later the artifacts become thus obsolete, being eventually disabled, archived and/or removed from the infrastructure.
Many artifacts require a considerable number of resources for their creation and maintenance over time. Sometimes the costs can be considerably higher than the benefits brought, especially when the data or the infrastructure are used for a narrow scope, though there can be other components that need to be considered in the bigger picture. Having a report or visualization one can use when needed can have an important impact on the business in correcting issues, sizing opportunities or filling the knowledge gaps.
Even if it’s challenging to quantify the costs associated with the loss of opportunities rooted in the lack of data, respectively information, the amounts can be considerable high, greater even than building a whole BI infrastructure. Organization’s agility in addressing the important gaps can make a considerable difference, at least in theory. Having the resources that can be pulled on demand can give organizations the needed competitive boost. Internal or external resources can be used altogether, though, pragmatically speaking, there will be always a gap between demand and supply of knowledgeable resources.
The gap in BI artefacts can be addressed nowadays by AI-driven tools, which have the theoretical potential of shortening the gap between needs and the availability of solutions, respectively a set of answers that can be used in the process. Of course, the processes of sense-making and discovery are not that simple as we’d like, though it’s a considerable step forward.
Having the possibility of asking questions in natural language and guiding the exploration process to create visualizations and other artifacts using prompt engineering and other AI-enabled methods offers new possibilities and opportunities that at least some organizations started exploring already. This however presumes the existence of an infrastructure on which the needed foundation can be built upon, the knowledge required to bridge the gap, respectively the resources required in the process.
It must be stressed out that the exploration processes may bring no sensible benefits, at least no immediately, and the whole process depends on organizations’ capabilities of identifying and sizing the respective opportunities. Therefore, even if there are recipes for success, each organization must identify what matters and how to use technologies and the available infrastructure to bridge the gap.
Ideally to make progress organizations need besides the financial resources the required skillset, a set of projects that support learning and value creation, respectively the design and execution of a business strategy that addresses the steps ahead. Each of these aspects implies risks and opportunities altogether. It will be a test of maturity for many organizations. It will be interesting to see how many organizations can handle the challenge, respectively how much past successes or failures will weigh in the balance.
AI offers a set of capabilities and opportunities, however the chance of exploring and failing fast is of great importance. AI is an enabler and not a magic wand, no matter what is preached in technical workshops! Even if progress follows an exponential trajectory, it took us more than half of century from the first steps until now and probably many challenges must be still overcome.
The future looks interesting enough to be pursued, though are organizations capable to size the opportunities, respectively to overcome the challenges ahead? Are organizations capable of supporting the effort without neglecting the other priorities?
08 April 2024
🧭Business Intelligence: Why Data Projects Fail to Deliver Real-Life Impact (Part III: Failure through the Looking Glass)
![]() |
Business Intelligence Series |
There’s a huge volume of material available on project failure – resources that document why individual projects failed, why in general projects fail, why project members, managers and/or executives think projects fail, and there seems to be no other more rewarding activity at the end of a project than to theorize why a project failed, the topic culminating occasionally with the blaming game. Success may generate applause, though it's failure that attracts and stirs the most waves (irony, disapproval, and other similar behavior) and everybody seems to be an expert after the consumed endeavor.
The mere definition of a project failure – not fulfilling project’s objectives within the set budget and timeframe - is a misnomer because budgets and timelines are estimated based on the information available at the beginning of the project, the amount of uncertainty for many projects being considerable, and data projects are no exceptions from it. The higher the uncertainty the less probable are the two estimates. Even simple projects can reveal uncertainty especially when the broader context of the projects is considered.
Even if it’s not a common practice, one way to cope with uncertainty is to add a tolerance for the estimates, though even this practice probably will not always accommodate the full extent of the unknown as the tolerances are usually small. The general expectation is to have an accurate and precise landing, which for big or exploratory projects is seldom possible!
Moreover, the assumptions under which the estimates hold are easily invalidated in praxis – resources’ availability, first time right, executive’s support to set priorities, requirements’ quality, technologies’ maturity, etc. If one looks beyond the reasons why projects fail in general, quite often the issues are more organizational than technological, the lack of knowledge and experience being some of the factors.
Conversely, many projects will not get approved if the estimates don’t look positive, and therefore people are pressured in one way or another to make the numbers fit the expectations. Some projects, given their importance, need to be done even if the numbers don’t look good or can’t be quantified correctly. Other projects represent people’s subsistence on the job, respectively people's self-occupation to create motion, though they can occasionally have also a positive impact for the organizations. These kinds of aspects almost never make it in statistics or surveys. Neither do the big issues people are afraid to talk about. Where to consider that in the light of politics and office’s grapevine the facts get distorted!
Data projects reflect all the symptoms of failure projects have in general, though when words like AI, Statistics or Machine Learning are used, the chances for failure are even higher given that the respective fields require a higher level of expertise, the appropriate use of technologies and adherence to the scientific process for the results to be valid. If projects can benefit from general recipes, respectively established procedures and methods, their range of applicability decreases when the mentioned areas are involved.
Many data projects have an exploratory nature – seeing what’s possible - and therefore a considerable percentage will not reach production. Moreover, even those that reach that far might arrive to be stopped or discarded sooner or later if they don’t deliver the expected value, and probably many of the models created in the process are biased, irrelevant, or incorrectly apply the theory. Where to add that the mere use of tools and algorithms is not Data Science or Data Analysis.
The challenge for many data projects is to identify which Project Management (PM) best practices to consider. Following all or no practices at all just increases the risks of failure!
Previous Post <<||>> Next Post
About Me
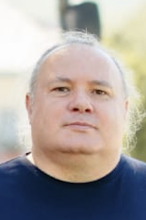
- Adrian
- Koeln, NRW, Germany
- IT Professional with more than 25 years experience in IT in the area of full life-cycle of Web/Desktop/Database Applications Development, Software Engineering, Consultancy, Data Management, Data Quality, Data Migrations, Reporting, ERP implementations & support, Team/Project/IT Management, etc.