![]() |
Data Visualization Series |
A Software Engineer and data professional's blog on SQL, data, databases, data architectures, data management, programming, Software Engineering, Project Management, ERP implementation and other IT related topics.
Pages
- 🏠Home
- 🗃️Definitions
- 🔢SQL Server
- 🎞️SQL Server: VoD
- 🏭Fabric
- 🎞️Fabric: VoD
- ⚡Power BI
- 🎞️Power BI: VoD
- 📚Data
- 📚Engineering
- 📚Management
- 📚SQL Server
- 🎞️D365: VoD
- 📚Systems Thinking
- ✂...Quotes
- 🧾D365: GL
- 💸D365: AP
- 💰D365: AR
- 🏠D365: FA
- 👥D365: HR
- ⛓️D365: SCM
- 🔤Acronyms
- 🪢Experts
- 🗃️Quotes
- 🔠Dataviz & BI
- 🔠D365
- 🔠Fabric
- 🔠Engineering
- 🔠Management
- 🔡Glossary
- 🌐Resources
- 🏺Dataviz
- 🗺️Social
- 📅Events
- ℹ️ About
14 December 2024
🧭💹Business Intelligence: Perspectives (Part 21: Data Visualization Revised)
20 March 2021
🧭Business Intelligence: New Technologies, Old Challenges (Part II - ETL vs. ELT)
Data lakes and similar cloud-based repositories drove the requirement of loading the raw data before performing any transformations on the data. At least that’s the approach the new wave of ELT (Extract, Load, Transform) technologies use to handle analytical and data integration workloads, which is probably recommendable for the mentioned cloud-based contexts. However, ELT technologies are especially relevant when is needed to handle data with high velocity, variance, validity or different value of truth (aka big data). This because they allow processing the workloads over architectures that can be scaled with workloads’ demands.
This is probably the most important aspect, even if there can
be further advantages, like using built-in connectors to a wide range of sources
or implementing complex data flow controls. The ETL (Extract, Transform, Load) tools
have the same capabilities, maybe reduced to certain data sources, though their
newer versions seem to bridge the gap.
One of the most stressed advantages of ELT is the possibility
of having all the (business) data in the repository, though these are not
technological advantages. The same can be obtained via ETL tools, even if this might
involve upon case a bigger effort, effort depending on the functionality existing
in each tool. It’s true that ETL solutions have a narrower scope by loading a subset
of the available data, or that transformations are made before loading the data,
though this depends on the scope considered while building the data warehouse or
data mart, respectively the design of ETL packages, and both are a matter of choice,
choices that can be traced back to business requirements or technical best practices.
Some of the advantages seen are context-dependent – the context
in which the technologies are put, respectively the problems are solved. It is often
imputed to ETL solutions that the available data are already prepared (aggregated,
converted) and new requirements will drive additional effort. On the other side,
in ELT-based solutions all the data are made available and eventually further transformed,
but also here the level of transformations made depends on specific requirements.
Independently of the approach used, the data are still available if needed, respectively
involve certain effort for further processing.
Building usable and reliable data models is dependent on good
design, and in the design process reside the most important challenges. In theory,
some think that in ETL scenarios the design is done beforehand though that’s not
necessarily true. One can pull the raw data from the source and build the data models
in the target repositories.
Data conversion and cleaning is needed under both approaches.
In some scenarios is ideal to do this upfront, minimizing the effect these processes
have on data’s usage, while in other scenarios it’s helpful to address them later
in the process, with the risk that each project will address them differently. This
can become an issue and should be ideally addressed by design (e.g. by building
an intermediate layer) or at least organizationally (e.g. enforcing best practices).
Advancing that ELT is better just because the data are true
(being in raw form) can be taken only as a marketing slogan. The degree of truth
data has depends on the way data reflects business’ processes and the way data are
maintained, while their quality is judged entirely on their intended use. Even if
raw data allow more flexibility in handling the various requests, the challenges
involved in processing can be neglected only under the consequences that follow
from this.
Looking at the analytics and data integration cloud-based technologies,
they seem to allow both approaches, thus building optimal solutions relying on professionals’
wisdom of making appropriate choices.
Previous Post <<||>>Next Post
02 December 2018
🔭Data Science: All Molels Are Wrong (Just the Quotes)
“[…] no models are [true] = not even the Newtonian laws. When you construct a model you leave out all the details which you, with the knowledge at your disposal, consider inessential. […] Models should not be true, but it is important that they are applicable, and whether they are applicable for any given purpose must of course be investigated. This also means that a model is never accepted finally, only on trial.” (Georg Rasch, “Probabilistic Models for Some Intelligence and Attainment Tests”, 1960)
“Celestial navigation is based on the premise that the Earth is the center of the universe. The premise is wrong, but the navigation works. An incorrect model can be a useful tool.” (R A J Phillips, “A Day in the Life of Kelvin Throop”, Analog Science Fiction and Science Fact, Vol. 73 No. 5, 1964)
“Since all models are wrong the scientist cannot obtain a ‘correct’ one by excessive elaboration. On the contrary following William of Occam he should seek an economical description of natural phenomena. Just as the ability to devise simple but evocative models is the signature of the great scientist so overelaboration and overparameterization is often the mark of mediocrity.” (George Box, “Science and Statistics", Journal of the American Statistical Association 71, 1976)
“A model of the universe does not require faith, but a telescope. If it is wrong, it is wrong.” (Paul C W Davies, “Space and Time in the Modern Universe”, 1977)
"Competent scientists do not believe their own models or theories, but rather treat them as convenient fictions. […] The issue to a scientist is not whether a model is true, but rather whether there is another whose predictive power is enough better to justify movement from today's fiction to a new one." (Steve Vardeman," Comment", Journal of the American Statistical Association 82, 1987)
“The fact that [the model] is an approximation does not necessarily detract from its usefulness because models are approximations. All models are wrong, but some are useful.” (George Box, 1987)“[…] it does not seem helpful just to say that all models are wrong. The very word model implies simplification and idealization. The idea that complex physical, biological or sociological systems can be exactly described by a few formulae is patently absurd. The construction of idealized representations that capture important stable aspects of such systems is, however, a vital part of general scientific analysis and statistical models, especially substantive ones, do not seem essentially different from other kinds of model.” (Sir David Cox, "Comment on ‘Model uncertainty, data mining and statistical inference’", Journal of the Royal Statistical Society, Series A 158, 1995)
“I do not know that my view is more correct; I do not even think that ‘right’ and ‘wrong’ are good categories for assessing complex mental models of external reality - for models in science are judged [as] useful or detrimental, not as true or false.” (Stephen Jay Gould, “Dinosaur in a Haystack: Reflections in Natural History”, 1995)
“No matter how beautiful the whole model may be, no matter how naturally it all seems to hang together now, if it disagrees with experiment, then it is wrong.” (John Gribbin, “Almost Everyone’s Guide to Science”, 1999)
“A model is a simplification or approximation of reality and hence will not reflect all of reality. […] Box noted that ‘all models are wrong, but some are useful’. While a model can never be ‘truth’, a model might be ranked from very useful, to useful, to somewhat useful to, finally, essentially useless.” (Kenneth P Burnham & David R Anderson, “Model Selection and Multimodel Inference: A Practical Information-Theoretic Approach” 2nd Ed., 2005)
“In general, when building statistical models, we must not forget that the aim is to understand something about the real world. Or predict, choose an action, make a decision, summarize evidence, and so on, but always about the real world, not an abstract mathematical world: our models are not the reality - a point well made by George Box in his oft-cited remark that “all models are wrong, but some are useful”. (David Hand, "Wonderful examples, but let's not close our eyes", Statistical Science 29, 2014)
About Me
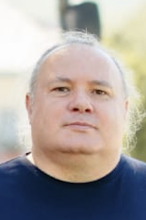
- Adrian
- Koeln, NRW, Germany
- IT Professional with more than 25 years experience in IT in the area of full life-cycle of Web/Desktop/Database Applications Development, Software Engineering, Consultancy, Data Management, Data Quality, Data Migrations, Reporting, ERP implementations & support, Team/Project/IT Management, etc.