A Software Engineer and data professional's blog on SQL, data, databases, data architectures, data management, programming, Software Engineering, Project Management, ERP implementation and other IT related topics.
Pages
- 🏠Home
- 🗃️Posts
- 🗃️Definitions
- 🔢SQL Server
- 🎞️SQL Server: VoD
- 🏭Fabric
- 🎞️Fabric: VoD
- ⚡Power BI
- 🎞️Power BI: VoD
- 📚Data
- 📚Engineering
- 📚Management
- 📚SQL Server
- 🎞️D365: VoD
- 📚Systems Thinking
- ✂...Quotes
- 🧾D365: GL
- 💸D365: AP
- 💰D365: AR
- 🏠D365: FA
- 👥D365: HR
- ⛓️D365: SCM
- 🔤Acronyms
- 🪢Experts
- 🗃️Quotes
- 🔠Dataviz & BI
- 🔠D365
- 🔠Fabric
- 🔠Engineering
- 🔠Management
- 🔡Glossary
- 🌐Resources
- 🏺Dataviz
- 🗺️Social
- 📅Events
- ℹ️ About
06 January 2016
♜Strategic Management: Governance (Definitions)
05 January 2016
♜Strategic Management: Roadmap (Definitions)
"An abstracted plan for business or technology change, typically operating across multiple disciplines over multiple years." (David Lyle & John G Schmidt, "Lean Integration", 2010)
"Techniques that capture market trends, product launches, technology development, and competence building over time in a multilayer, consistent framework." (Gina C O'Connor & V K Narayanan, "Encyclopedia of Technology and Innovation Management", 2010)
"Defines the actions required to move from current to future (target) state. Similar to a high-level project plan." (DAMA International, "The DAMA Dictionary of Data Management", 2011)
[portfolio roadmap:] "A document that provides the high-level strategic direction and portfolio information in a chronological fashion for portfolio management and ensures dependencies within the portfolio are established and evaluated." (Project Management Institute, "The Standard for Portfolio Management" 3rd Ed., 2012)
"Forward-looking plans intended to be taken by the security program over the foreseeable future." (Mark Rhodes-Ousley, "Information Security: The Complete Reference" 2nd Ed., 2013)
"Within the context of business analytics, a defined set of staged initiatives that deliver tactical returns while moving the team toward strategic outcomes." (Evan Stubbs, "Delivering Business Analytics: Practical Guidelines for Best Practice", 2013)
"High-level action plan for change that will involve several facets of the enterprise (business, organization, technical)." (Gilbert Raymond & Philippe Desfray, "Modeling Enterprise Architecture with TOGAF", 2014)
"An action plan that matches the organization's business goals with specific technology solutions in order to help meet those goals." (David K Pham, "From Business Strategy to Information Technology Roadmap", 2016)
"The Roadmap is a schedule of events and Milestones that communicate planned Solution deliverables over a timeline. It includes commitments for the planned, upcoming Program Increment (PI) and offers visibility into the deliverables forecasted for the next few PIs." (Dean Leffingwell, "SAFe 4.5 Reference Guide: Scaled Agile Framework for Lean Enterprises" 2nd Ed., 2018)
"A product roadmap is a visual summary of a product’s direction to facilitate communication with customers, prospects, partners, and internal stakeholders." (Pendo) [source]
"A Roadmap is a plan to progress toward a set of defined goals. Depending on the purpose of the Roadmap, it may be either high-level or detailed. In terms of Enterprise Architecture, roadmaps are usually developed as abstracted plans for business or technology changes, typically operating across multiple disciplines over multiple years." (Orbus Software)
"A roadmap is a strategic plan that defines a goal or desired outcome and includes the major steps or milestones needed to reach it." (ProductPlan) [source]
04 January 2016
♜Strategic Management: Risk Mitigation (Definitions)
"A planning process to identify, prevent, remove, or reduce risk if it occurs and define actions to limit the severity/impact of a risk, should it occur." (Lynne Hambleton, "Treasure Chest of Six Sigma Growth Methods, Tools, and Best Practices", 2007)
"The act of developing advance plans or taking immediate actions to minimize, or prevent known or unknown events (risks) from adversely impacting a strategy or business objective." (Steven G Haines, "The Product Manager's Desk Reference", 2008)
"A risk response strategy whereby the project team acts to reduce the probability of occurrence or impact of a threat. " (Project Management Institute, "The Standard for Portfolio Management" 3rd Ed., 2012)
"Reducing a risk by controlling its likelihood, its cost, or its threats, through the use of security measures designed to provide these controls." (Mark Rhodes-Ousley, "Information Security: The Complete Reference, Second Edition, 2nd Ed.", 2013)
"The process through which decisions are reached and protective measures are implemented for reducing risk to, or maintaining risks within, specified levels." (ISTQB)
03 January 2016
♜Strategic Management: Business Strategy (Definitions)
♜Strategic Management: Balanced Scorecard (Definitions)
02 January 2016
♜Strategic Management: Risk Management (Definitions)
"An organized, analytic process to identify what might cause harm or loss (identify risks); to assess and quantify the identified risks; and to develop and, if needed, implement an appropriate approach to prevent or handle causes of risk that could result in significant harm or loss." (Sandy Shrum et al, "CMMI: Guidelines for Process Integration and Product Improvement", 2003)
"The organized, analytic process to identify future events (risks) that might cause harm or loss, assess and quantify the identified risks, and decide if, how, and when to prevent or reduce the risk. Also includes the implementation of mitigation actions at the appropriate times." (Richard D Stutzke, "Estimating Software-Intensive Systems: Projects, Products, and Processes", 2005)
"Identifying a situation or problem that may put specific plans or outcomes in jeopardy, and then organizing actions to mitigate it." (Teri Lund & Susan Barksdale, "10 Steps to Successful Strategic Planning", 2006)
"The process of identifying hazards of property insured; the casualty contemplated in a specific contract of insurance; the degree of hazard; a specific contingency or peril. Generally not the same as security management, but may be related in concerns and activities. Work is done by a risk manager." (Robert McCrie, "Security Operations Management" 2nd Ed., 2006)
"Systematic application of procedures and practices to the tasks of identifying, analyzing, prioritizing, and controlling risk." (Tilo Linz et al, "Software Testing Practice: Test Management", 2007)
"Risk management is a continuous process to be performed throughout the entire life of a project, and an important part of project management activities. The objective of risk management is to identify and prevent risks, to reduce their probability of occurrence, or to mitigate the effects in case of risk occurrence." (Lars Dittmann et al, "Automotive SPICE in Practice", 2008)
"A structured process for managing risk." (David G Hill, "Data Protection: Governance, Risk Management, and Compliance", 2009)
"The process organizations employ to reduce different types of risks. A company manages risk to avoid losing money, protect against breaking government or regulatory body rules, or even assure that adverse weather does not interrupt the supply chain." (Tony Fisher, "The Data Asset", 2009)
"Systematic application of procedures and practices to the tasks of identifying, analyzing, prioritizing, and controlling risk." (IQBBA, "Standard glossary of terms used in Software Engineering", 2011)
"The process of identifying what can go wrong, determining how to respond to risks should they occur, monitoring a project for risks that do occur, and taking steps to respond to the events that do occur." (Bonnie Biafore, "Successful Project Management: Applying Best Practices and Real-World Techniques with Microsoft® Project", 2011)
"Risk management is using managerial resources to integrate risk identification, risk assessment, risk prioritization, development of risk-handling strategies, and mitigation of risk to acceptable levels (ASQ)." (Laura Sebastian-Coleman, "Measuring Data Quality for Ongoing Improvement ", 2012)
"The process of identifying negative and positive risks to a project, analyzing the likelihood and impact of those risks, planning responses to higher priority risks, and tracking risks." (Bonnie Biafore & Teresa Stover, "Your Project Management Coach: Best Practices for Managing Projects in the Real World", 2012)
"A policy of determining the greatest potential failure associated with a project." (James Robertson et al, "Complete Systems Analysis: The Workbook, the Textbook, the Answers", 2013)
"Controlling vulnerabilities, threats, likelihood, loss, or impact with the use of security measures. See also risk, threat, and vulnerability." (Mark Rhodes-Ousley, "Information Security: The Complete Reference, Second Edition" 2nd Ed., 2013)
"A process to identify, assess, manage, and control potential events or situations to provide reasonable assurance regarding the achievement of the organization's objectives." (Sally-Anne Pitt, "Internal Audit Quality", 2014)
"Managing the financial impacts of unusual events." (Manish Agrawal, "Information Security and IT Risk Management", 2014)
"Systematic application of policies, procedures, methods and practices to the tasks of identifying, analysing, evaluating, treating and monitoring risk." (Chartered Institute of Building, "Code of Practice for Project Management for Construction and Development, 5th Ed.", 2014)
"The coordinated activities to direct and control an organisation with regard to risk." (David Sutton, "Information Risk Management: A practitioner’s guide", 2014)
"The process of reducing risk to an acceptable level by implementing security controls. Organizations implement risk management programs to identify risks and methods to reduce it. The risk that remains after risk has been mitigated to an acceptable level is residual risk." (Darril Gibson, "Effective Help Desk Specialist Skills", 2014)
"Risk management is a structured approach to monitoring, measuring, and managing exposures to reduce the potential impact of an uncertain happening." (Christopher Donohue et al, "Foundations of Financial Risk: An Overview of Financial Risk and Risk-based Financial Regulation, 2nd Ed", 2015)
"Systematic application of procedures and practices to the tasks of identifying, analyzing, prioritizing, and controlling risk. " (ISTQB, "Standard Glossary", 2015)
"The practice of identifying, assessing, controlling, and mitigating risks. Techniques to manage risk include avoiding, transferring, mitigating, and accepting the risk." (Weiss, "Auditing IT Infrastructures for Compliance, 2nd Ed", 2015)
"The discipline and methods used to quantify, track, and reduce where possible various types of defined risk." (Gregory Lampshire, "The Data and Analytics Playbook", 2016)
"The process of identifying individual risks, understanding and analyzing them, and then managing them." (Paul H Barshop, "Capital Projects", 2016)
"Coordinated activities to direct and control an organization with regard to risk." (William Stallings, "Effective Cybersecurity: A Guide to Using Best Practices and Standards", 2018)
"Process of identifying and monitoring business risks in a manner that offers a risk/return relationship that is acceptable to an entity's operating philosophy." (Tom Klammer, "Statement of Cash Flows: Preparation, Presentation, and Use", 2018)
"Coordinated activities to direct and control an organisation with regard to risk." (ISO Guide 73:2009)
"Risk management is the identification, assessment and prioritisation of risks [...] followed by coordinated and economical application of resources to minimise, monitor and control the probability and/or impact of unfortunate events or to maximise the realisation of opportunities." (David Sutton, "Information Risk Management: A practitioner’s guide", 2014)
♜Strategic Management: Enterprise Architecture (Definitions)
"[Enterprise Architecture is] the set of descriptive representations (i. e., models) that are relevant for describing an Enterprise such that it can be produced to management's requirements (quality) and maintained over the period of its useful life. (John Zachman, 1987)
"An enterprise architecture is an abstract summary of some organizational component's design. The organizational strategy is the basis for deciding where the organization wants to be in three to five years. When matched to the organizational strategy, the architectures provide the foundation for deciding priorities for implementing the strategy." (Sue A Conger, "The new software engineering", 1994)
"An enterprise architecture is a snapshot of how an enterprise operates while performing its business processes. The recognition of the need for integration at all levels of an organisation points to a multi-dimensional framework that links both the business processes and the data requirements." (John Murphy & Brian Stone [Eds.], 1995)
"The Enterprise Architecture is the explicit description of the current and desired relationships among business and management process and information technology. It describes the 'target' situation which the agency wishes to create and maintain by managing its IT portfolio." (Franklin D Raines, 1997)
"Enterprise architecture is a family of related architecture components. This include information architecture, organization and business process architecture, and information technology architecture. Each consists of architectural representations, definitions of architecture entities, their relationships, and specification of function and purpose. Enterprise architecture guides the construction and development of business organizations and business processes, and the construction and development of supporting information systems." (Gordon B Davis, "The Blackwell encyclopedic dictionary of management information systems", 1999)
"Enterprise architecture is a holistic representation of all the components of the enterprise and the use of graphics and schemes are used to emphasize all parts of the enterprise, and how they are interrelated." (Gordon B Davis," The Blackwell encyclopedic dictionary of management information systems", 1999)
"Enterprise Architecture is the discipline whose purpose is to align more effectively the strategies of enterprises together with their processes and their resources (business and IT)." (Alain Wegmann, "On the systemic enterprise architecture methodology", 2003)
"An enterprise architecture is a blueprint for organizational change defined in models [using words, graphics, and other depictions] that describe (in both business and technology terms) how the entity operates today and how it intends to operate in the future; it also includes a plan for transitioning to this future state." (US Government Accountability Office, "Enterprise Architecture: Leadership Remains Key to Establishing and Leveraging Architectures for Organizational Transformation", GAO-06-831, 2006)
"Enterprise architecture is the organizing logic for business processes and IT infrastructure reflecting the integration and standardization requirements of a company's operation model." (Jeanne W. Ross et al, "Enterprise architecture as strategy: creating a foundation for business", 2006)
"Enterprise-architecture is the integration of everything the enterprise is and does." (Tom Graves, "Real Enterprise-Architecture : Beyond IT to the whole enterprise", 2007)
"Enterprise architecture is the organizing logic for business processes and IT infrastructure reflecting the integration and standardization requirements of the company's operating model. The operating model is the desired state of business process integration and business process standardization for delivering goods and services to customers." (Peter Weill, "Innovating with Information Systems Presentation", 2007)
"Enterprise architecture is the process of translating business vision and strategy into effective enterprise change by creating, communicating and improving the key requirements, principles and models that describe the enterprise's future state and enable its evolution. The scope of the enterprise architecture includes the people, processes, information and technology of the enterprise, and their relationships to one another and to the external environment. Enterprise architects compose holistic solutions that address the business challenges of the enterprise and support the governance needed to implement them." (Anne Lapkin et al, "Gartner Clarifies the Definition of the Term 'Enterprise Architecture", 2008)
"Enterprise architecture [is] a coherent whole of principles, methods, and models that are used in the design and realisation of an enterprise's organisational structure, business processes, information systems, and infrastructure." (Marc Lankhorst, "Enterprise Architecture at Work: Modelling, Communication and Analysis", 2009)
"Enterprise architecture (EA) is the definition and representation of a high-level view of an enterprise‘s business processes and IT systems, their interrelationships, and the extent to which these processes and systems are shared by different parts of the enterprise. EA aims to define a suitable operating platform to support an organisation‘s future goals and the roadmap for moving towards this vision." (Toomas Tamm et al, "How Does Enterprise Architecture Add Value to Organisations?", Communications of the Association for Information Systems Vol. 28 (10), 2011)
"Enterprise architecture (EA) is a discipline for proactively and holistically leading enterprise responses to disruptive forces by identifying and analyzing the execution of change toward desired business vision and outcomes. EA delivers value by presenting business and IT leaders with signature-ready recommendations for adjusting policies and projects to achieve target business outcomes that capitalize on relevant business disruptions. EA is used to steer decision making toward the evolution of the future state architecture." (Gartner)
"Enterprise Architecture [...] is a way of thinking enabled by patterns, frameworks, standards etc. essentially seeking to align both the technology ecosystem and landscape with the business trajectory driven by both the internal and external forces." (Daljit R Banger)
01 January 2016
♜Strategic Management: Strategy (Definitions)
31 December 2015
🪙Business Intelligence: Data Fabric (Just the Quotes)
"Data architects often turn to graphs because they are flexible enough to accommodate multiple heterogeneous representations of the same entities as described by each of the source systems. With a graph, it is possible to associate underlying records incrementally as data is discovered. There is no need for big, up-front design, which serves only to hamper business agility. This is important because data fabric integration is not a one-off effort and a graph model remains flexible over the lifetime of the data domains." (Jesús Barrasa et al, "Knowledge Graphs: Data in Context for Responsive Businesses", 2021)
"Data fabrics are general-purpose, organization-wide data access interfaces that offer a connected view of the integrated domains by combining data stored in a local graph with data retrieved on demand from third-party systems. Their job is to provide a sophisticated index and integration points so that they can curate data across silos, offering consistent capabilities regardless of the underlying store (which might or might not be graph based) […]." (Jesús Barrasa et al, "Knowledge Graphs: Data in Context for Responsive Businesses", 2021)
"A Data Fabric has its focus more on the architectural underpinning, technical capabilities, and intelligent analysis to produce active metadata supporting a smarter, AI-infused system to orchestrate various data integration styles, enabling trusted and reusable data in a hybrid cloud landscape to be consumed by humans, applications, or other downstream systems." (Eberhard Hechler et al, "Data Fabric and Data Mesh Approaches with AI", 2023)
"Data Fabric’s building blocks represent groupings of different components and characteristics. They are high-level blocks that describe a package of capabilities that address specific business needs. The building blocks are Data Governance and its knowledge layer, Data Integration, and Self-Service." (Sonia Mezzetta, "Principles of Data Fabric: Become a data-driven organization by implementing Data Fabric solutions efficiently", 2023)
"Data Fabric is a composable architecture made up of different tools, technologies, and systems. It has an active metadata and event-driven design that automates Data Integration while achieving interoperability. Data Governance, Data Privacy, Data Protection, and Data Security are paramount to its design and to enable Self-Service data sharing. The following figure summarizes the different characteristics that constitute a Data Fabric design." (Sonia Mezzetta, "Principles of Data Fabric: Become a data-driven organization by implementing Data Fabric solutions efficiently", 2023)
"Data Fabric is a distributed data architecture that connects scattered data across tools and systems with the objective of providing governed access to fit-for-purpose data at speed. Data Fabric focuses on Data Governance, Data Integration, and Self-Service data sharing. It leverages a sophisticated active metadata layer that captures knowledge derived from data and its operations, data relationships, and business context. Data Fabric continuously analyzes data management activities to recommend value-driven improvements. Data Fabric works with both centralized and decentralized data systems and supports diverse operational models." (Sonia Mezzetta, "Principles of Data Fabric: Become a data-driven organization by implementing Data Fabric solutions efficiently", 2023)
"Enterprises have difficulties in interpreting new concepts like the data mesh and data fabric, because pragmatic guidance and experiences from the field are missing. In addition to that, the data mesh fully embraces a decentralized approach, which is a transformational change not only for the data architecture and technology, but even more so for organization and processes. This means the transformation cannot only be led by IT; it’s a business transformation as well." (Piethein Strengholt, "Data Management at Scale: Modern Data Architecture with Data Mesh and Data Fabric" 2nd Ed., 2023)
"Gaining more insight into data, simplifying data access, enabling shopping-for-data, augmenting traditional data governance, generating active metadata, and accelerating development of products and services are enabled by infusing AI into the Data Fabric architecture. An AI-infused Data Fabric is not only leveraging AI but also likewise an architecture to manage and deal with AI artefacts, including AI models, pipelines, etc." (Eberhard Hechler et al, "Data Fabric and Data Mesh Approaches with AI", 2023)
"The data fabric is an approach that addresses today’s data management and scalability challenges by adding intelligence and simplifying data access using self-service. In contrast to the data mesh, it focuses more on the technology layer. It’s an architectural vision using unified metadata with an end-to-end integrated layer (fabric) for easily accessing, integrating, provisioning, and using data." (Piethein Strengholt, "Data Management at Scale: Modern Data Architecture with Data Mesh and Data Fabric" 2nd Ed., 2023)
"At its core, a data fabric is an architectural framework, designed to be employed within one or more domains inside a data mesh. The data mesh, however, is a holistic concept, encompassing technology, strategies, and methodologies." (James Serra, "Deciphering Data Architectures", 2024)
"It is very important to understand that data mesh is a concept, not a technology. It is all about an organizational and cultural shift within companies. The technology used to build a data mesh could follow the modern data warehouse, data fabric, or data lakehouse architecture - or domains could even follow different architectures. (James Serra, "Deciphering Data Architectures", 2024)
30 December 2015
🪙Business Intelligence: Complexity (Just the Quotes)
"The more complex the shape of any object. the more difficult it is to perceive it. The nature of thought based on the visual apprehension of objective forms suggests, therefore, the necessity to keep all graphics as simple as possible. Otherwise, their meaning will be lost or ambiguous, and the ability to convey the intended information and to persuade will be inhibited." (Robert Lefferts, "Elements of Graphics: How to prepare charts and graphs for effective reports", 1981)
"Largeness comes in different forms and has many different effects. Whereas some tasks remain easy, others become obstinately difficult. Largeness is not just an increase in dataset size. [...] Largeness may mean more complexity - more variables, more detail (additional categories, special cases), and more structure (temporal or spatial components, combinations of relational data tables). Again this is not so much of a problem with small datasets, where the complexity will be by definition limited, but becomes a major problem with large datasets. They will often have special features that do not fit the standard case by variable matrix structure well-known to statisticians." (Antony Unwin et al [in "Graphics of Large Datasets: Visualizing a Million"], 2006)
"Once these different measures of performance are consolidated into a single number, that statistic can be used to make comparisons […] The advantage of any index is that it consolidates lots of complex information into a single number. We can then rank things that otherwise defy simple comparison […] Any index is highly sensitive to the descriptive statistics that are cobbled together to build it, and to the weight given to each of those components. As a result, indices range from useful but imperfect tools to complete charades." (Charles Wheelan, "Naked Statistics: Stripping the Dread from the Data", 2012)
"The urge to tinker with a formula is a hunger that keeps coming back. Tinkering almost always leads to more complexity. The more complicated the metric, the harder it is for users to learn how to affect the metric, and the less likely it is to improve it." (Kaiser Fung, "Numbersense: How To Use Big Data To Your Advantage", 2013)
"Any presentation of data, whether a simple calculated metric or a complex predictive model, is going to have a set of assumptions and choices that the producer has made to get to the output. The more that these can be made explicit, the more the audience of the data will be open to accepting the message offered by the presenter." (Zach Gemignani et al, "Data Fluency", 2014)
"Decision trees are also considered nonparametric models. The reason for this is that when we train a decision tree from data, we do not assume a fixed set of parameters prior to training that define the tree. Instead, the tree branching and the depth of the tree are related to the complexity of the dataset it is trained on. If new instances were added to the dataset and we rebuilt the tree, it is likely that we would end up with a (potentially very) different tree." (John D Kelleher et al, "Fundamentals of Machine Learning for Predictive Data Analytics: Algorithms, Worked Examples, and Case Studies", 2015)
"When datasets are small, a parametric model may perform well because the strong assumptions made by the model - if correct - can help the model to avoid overfitting. However, as the size of the dataset grows, particularly if the decision boundary between the classes is very complex, it may make more sense to allow the data to inform the predictions more directly. Obviously the computational costs associated with nonparametric models and large datasets cannot be ignored. However, support vector machines are an example of a nonparametric model that, to a large extent, avoids this problem. As such, support vector machines are often a good choice in complex domains with lots of data." (John D Kelleher et al, "Fundamentals of Machine Learning for Predictive Data Analytics: Algorithms, Worked Examples, and Case Studies", 2015)
"The tension between bias and variance, simplicity and complexity, or underfitting and overfitting is an area in the data science and analytics process that can be closer to a craft than a fixed rule. The main challenge is that not only is each dataset different, but also there are data points that we have not yet seen at the moment of constructing the model. Instead, we are interested in building a strategy that enables us to tell something about data from the sample used in building the model." (Jesús Rogel-Salazar, "Data Science and Analytics with Python", 2017)
🪙Business Intelligence: Data Analysis (Just the Quotes)
"Typically, data analysis is messy, and little details clutter it. Not only confounding factors, but also deviant cases, minor problems in measurement, and ambiguous results lead to frustration and discouragement, so that more data are collected than analyzed. Neglecting or hiding the messy details of the data reduces the researcher's chances of discovering something new." (Edward R Tufte, "Data Analysis for Politics and Policy", 1974)
"A first analysis of experimental results should, I believe, invariably be conducted using flexible data analytical techniques - looking at graphs and simple statistics - that so far as possible allow the data to 'speak for themselves'. The unexpected phenomena that such a approach often uncovers can be of the greatest importance in shaping and sometimes redirecting the course of an ongoing investigation." (George Box, "Signal to Noise Ratios, Performance Criteria, and Transformations", Technometrics 30, 1988)
"Data analysis is an art practiced by individuals who are skilled at quantitative reasoning and have much experience in looking at numbers and detecting patterns in data. Usually these individuals have some background in statistics." (David Lubinsky, Daryl Pregibon , "Data analysis as search", Journal of Econometrics Vol. 38 (1–2), 1988)
"Like a detective, a data analyst will experience many dead ends, retrace his steps, and explore many alternatives before settling on a single description of the evidence in front of him." (David Lubinsky & Daryl Pregibon , "Data analysis as search", Journal of Econometrics Vol. 38 (1–2), 1988)
"Data analysis is rarely as simple in practice as it appears in books. Like other statistical techniques, regression rests on certain assumptions and may produce unrealistic results if those assumptions are false. Furthermore it is not always obvious how to translate a research question into a regression model." (Lawrence C Hamilton, "Regression with Graphics: A second course in applied statistics", 1991)
"Data analysis typically begins with straight-line models because they are simplest, not because we believe reality is inherently linear. Theory or data may suggest otherwise [...]" (Lawrence C Hamilton, "Regression with Graphics: A second course in applied statistics", 1991)
"Probabilistic inference is the classical paradigm for data analysis in science and technology. It rests on a foundation of randomness; variation in data is ascribed to a random process in which nature generates data according to a probability distribution. This leads to a codification of uncertainly by confidence intervals and hypothesis tests." (William S Cleveland, "Visualizing Data", 1993)
"Put simply, statistics is a range of procedures for gathering, organizing, analyzing and presenting quantitative data. […] Essentially […], statistics is a scientific approach to analyzing numerical data in order to enable us to maximize our interpretation, understanding and use. This means that statistics helps us turn data into information; that is, data that have been interpreted, understood and are useful to the recipient. Put formally, for your project, statistics is the systematic collection and analysis of numerical data, in order to investigate or discover relationships among phenomena so as to explain, predict and control their occurrence." (Reva B Brown & Mark Saunders, "Dealing with Statistics: What You Need to Know", 2008)
"Statistics is an integral part of the quantitative approach to knowledge. The field of statistics is concerned with the scientific study of collecting, organizing, analyzing, and drawing conclusions from data." (Kandethody M Ramachandran & Chris P Tsokos, "Mathematical Statistics with Applications in R" 2nd Ed., 2015)
"Too often there is a disconnect between the people who run a study and those who do the data analysis. This is as predictable as it is unfortunate. If data are gathered with particular hypotheses in mind, too often they (the data) are passed on to someone who is tasked with testing those hypotheses and who has only marginal knowledge of the subject matter. Graphical displays, if prepared at all, are just summaries or tests of the assumptions underlying the tests being done. Broader displays, that have the potential of showing us things that we had not expected, are either not done at all, or their message is not able to be fully appreciated by the data analyst." (Howard Wainer, Comment, Journal of Computational and Graphical Statistics Vol. 20(1), 2011)
"Data analysis and data mining are concerned with unsupervised pattern finding and structure determination in data sets. The data sets themselves are explicitly linked as a form of representation to an observational or otherwise empirical domain of interest. 'Structure' has long been understood as symmetry which can take many forms with respect to any transformation, including point, translational, rotational, and many others. Symmetries directly point to invariants, which pinpoint intrinsic properties of the data and of the background empirical domain of interest. As our data models change, so too do our perspectives on analysing data." (Fionn Murtagh, "Data Science Foundations: Geometry and Topology of Complex Hierarchic Systems and Big Data Analytics", 2018)
"[…] the data itself can lead to new questions too. In exploratory data analysis (EDA), for example, the data analyst discovers new questions based on the data. The process of looking at the data to address some of these questions generates incidental visualizations - odd patterns, outliers, or surprising correlations that are worth looking into further." (Danyel Fisher & Miriah Meyer, "Making Data Visual", 2018)
"Analysis is a two-step process that has an exploratory and an explanatory phase. In order to create a powerful data story, you must effectively transition from data discovery (when you’re finding insights) to data communication (when you’re explaining them to an audience). If you don’t properly traverse these two phases, you may end up with something that resembles a data story but doesn’t have the same effect. Yes, it may have numbers, charts, and annotations, but because it’s poorly formed, it won’t achieve the same results." (Brent Dykes, "Effective Data Storytelling: How to Drive Change with Data, Narrative and Visuals", 2019)
"While visuals are an essential part of data storytelling, data visualizations can serve a variety of purposes from analysis to communication to even art. Most data charts are designed to disseminate information in a visual manner. Only a subset of data compositions is focused on presenting specific insights as opposed to just general information. When most data compositions combine both visualizations and text, it can be difficult to discern whether a particular scenario falls into the realm of data storytelling or not." (Brent Dykes, "Effective Data Storytelling: How to Drive Change with Data, Narrative and Visuals", 2019)
"If the data that go into the analysis are flawed, the specific technical details of the analysis don’t matter. One can obtain stupid results from bad data without any statistical trickery. And this is often how bullshit arguments are created, deliberately or otherwise. To catch this sort of bullshit, you don’t have to unpack the black box. All you have to do is think carefully about the data that went into the black box and the results that came out. Are the data unbiased, reasonable, and relevant to the problem at hand? Do the results pass basic plausibility checks? Do they support whatever conclusions are drawn?" (Carl T Bergstrom & Jevin D West, "Calling Bullshit: The Art of Skepticism in a Data-Driven World", 2020)
"We all know that the numerical values on each side of an equation have to be the same. The key to dimensional analysis is that the units have to be the same as well. This provides a convenient way to keep careful track of units when making calculations in engineering and other quantitative disciplines, to make sure one is computing what one thinks one is computing. When an equation exists only for the sake of mathiness, dimensional analysis often makes no sense." (Carl T Bergstrom & Jevin D West, "Calling Bullshit: The Art of Skepticism in a Data-Driven World", 2020)
"Overall [...] everyone also has a need to analyze data. The ability to analyze data is vital in its understanding of product launch success. Everyone needs the ability to find trends and patterns in the data and information. Everyone has a need to ‘discover or reveal (something) through detailed examination’, as our definition says. Not everyone needs to be a data scientist, but everyone needs to drive questions and analysis. Everyone needs to dig into the information to be successful with diagnostic analytics. This is one of the biggest keys of data literacy: analyzing data." (Jordan Morrow, "Be Data Literate: The data literacy skills everyone needs to succeed", 2021)
[Murphy’s Laws of Analysis:] "(1) In any collection of data, the figures that are obviously correct contain errors. (2) It is customary for a decimal to be misplaced. (3) An error that can creep into a calculation, will. Also, it will always be in the direction that will cause the most damage to the calculation." (G C Deakly)
🪙Business Intelligence: Data Pipelines (Just the Quotes)
"Data Lake is a single window snapshot of all enterprise data in its raw format, be it structured, semi-structured, or unstructured. Starting from curating the data ingestion pipeline to the transformation layer for analytical consumption, every aspect of data gets addressed in a data lake ecosystem. It is supposed to hold enormous volumes of data of varied structures." (Saurabh Gupta et al, "Practical Enterprise Data Lake Insights", 2018
"The quality of data that flows within a data pipeline is as important as the functionality of the pipeline. If the data that flows within the pipeline is not a valid representation of the source data set(s), the pipeline doesn’t serve any real purpose. It’s very important to incorporate data quality checks within different phases of the pipeline. These checks should verify the correctness of data at every phase of the pipeline. There should be clear isolation between checks at different parts of the pipeline. The checks include checks like row count, structure, and data type validation." (Saurabh Gupta et al, "Practical Enterprise Data Lake Insights", 2018)
"For advanced analytics, a well-designed data pipeline is a prerequisite, so a large part of your focus should be on automation. This is also the most difficult work. To be successful, you need to stitch everything together." (Piethein Strengholt, "Data Management at Scale: Best Practices for Enterprise Architecture", 2020)
"A data pipeline is a series of transformation steps (functions) executed as the data flows from one step to another. Data mesh refrains from using pipelines as a top-level architectural paradigm and in between data products. The challenge with pipelines as currently used is that they don’t create clear interfaces, contracts, and abstractions that can be maintained easily as the pipeline complexity complexity grows. Due to lack of abstractions, single failure in the pipeline causes cascading failures." (Zhamak Dehghani, "Data Mesh: Delivering Data-Driven Value at Scale", 2021)
"Data lake architecture suffers from complexity and deterioration. It creates complex and unwieldy pipelines of batch or streaming jobs operated by a central team of hyper-specialized data engineers. It deteriorates over time. Its unmanaged datasets, which are often untrusted and inaccessible, provide little value. The data lineage and dependencies are obscured and hard to track." (Zhamak Dehghani, "Data Mesh: Delivering Data-Driven Value at Scale", 2021)
"Data mesh [...] reduces points of centralization that act as coordination bottlenecks. It finds a new way of decomposing the data architecture without slowing the organization down with synchronizations. It removes the gap between where the data originates and where it gets used and removes the accidental complexities - aka pipelines - that happen in between the two planes of data. Data mesh departs from data myths such as a single source of truth, or one tightly controlled canonical data model." (Zhamak Dehghani, "Data Mesh: Delivering Data-Driven Value at Scale", 2021)
"Data has historically been treated as a second-class citizen, as a form of exhaust or by-product emitted by business applications. This application-first thinking remains the major source of problems in today’s computing environments, leading to ad hoc data pipelines, cobbled together data access mechanisms, and inconsistent sources of similar-yet-different truths. Data mesh addresses these shortcomings head-on, by fundamentally altering the relationships we have with our data. Instead of a secondary by-product, data, and the access to it, is promoted to a first-class citizen on par with any other business service." (Adam Bellemare,"Building an Event-Driven Data Mesh: Patterns for Designing and Building Event-Driven Architectures", 2023)
"Gaining more insight into data, simplifying data access, enabling shopping-for-data, augmenting traditional data governance, generating active metadata, and accelerating development of products and services are enabled by infusing AI into the Data Fabric architecture. An AI-infused Data Fabric is not only leveraging AI but also likewise an architecture to manage and deal with AI artefacts, including AI models, pipelines, etc." (Eberhard Hechler et al, "Data Fabric and Data Mesh Approaches with AI", 2023)
26 December 2015
🪙Business Intelligence: Measurement (Just the Quotes)
"When you can measure what you are speaking about, and express it in numbers, you know something about it; but when you cannot express it in numbers, your knowledge is of a meager and unsatisfactory kind; it may be the beginning of knowledge, but you have scarcely in your thoughts advanced to the state of science.” (Lord Kelvin, "Electrical Units of Measurement", 1883)
“Of itself an arithmetic average is more likely to conceal than to disclose important facts; it is the nature of an abbreviation, and is often an excuse for laziness.” (Arthur Lyon Bowley, “The Nature and Purpose of the Measurement of Social Phenomena”, 1915)
“Science depends upon measurement, and things not measurable are therefore excluded, or tend to be excluded, from its attention.” (Arthur J Balfour, “Address”, 1917)
“It is important to realize that it is not the one measurement, alone, but its relation to the rest of the sequence that is of interest.” (William E Deming, “Statistical Adjustment of Data”, 1943)
“The purpose of computing is insight, not numbers […] sometimes […] the purpose of computing numbers is not yet in sight.” (Richard Hamming, “Numerical Methods for Scientists and Engineers”, 1962)
“A quantity like time, or any other physical measurement, does not exist in a completely abstract way. We find no sense in talking about something unless we specify how we measure it. It is the definition by the method of measuring a quantity that is the one sure way of avoiding talking nonsense...” (Hermann Bondi, “Relativity and Common Sense”, 1964)
“Measurement, we have seen, always has an element of error in it. The most exact description or prediction that a scientist can make is still only approximate.” (Abraham Kaplan, “The Conduct of Inquiry: Methodology for Behavioral Science”, 1964)
"A mature science, with respect to the matter of errors in variables, is not one that measures its variables without error, for this is impossible. It is, rather, a science which properly manages its errors, controlling their magnitudes and correctly calculating their implications for substantive conclusions." (Otis D Duncan, "Introduction to Structural Equation Models", 1975)
“Data in isolation are meaningless, a collection of numbers. Only in context of a theory do they assume significance […]” (George Greenstein, “Frozen Star”, 1983)
“The value of having numbers - data - is that they aren't subject to someone else's interpretation. They are just the numbers. You can decide what they mean for you.” (Emily Oster, “Expecting Better”, 2013)
25 December 2015
🪙Business Intelligence: Data Mesh (Just the quotes)
"Another myth is that we shall have a single source of truth for each concept or entity. […] This is a wonderful idea, and is placed to prevent multiple copies of out-of-date and untrustworthy data. But in reality it’s proved costly, an impediment to scale and speed, or simply unachievable. Data Mesh does not enforce the idea of one source of truth. However, it places multiple practices in place that reduces the likelihood of multiple copies of out-of-date data." (Zhamak Dehghani, "Data Mesh: Delivering Data-Driven Value at Scale", 2021)
"Data Mesh attempts to strike a balance between team autonomy and inter-term interoperability and collaboration, with a few complementary techniques. It gives domain teams autonomy to have control of their local decision making, such as choosing the best data model for their data products. While it uses the computational governance policies to impose a consistent experience across all data products; for example, standardizing on the data modeling language that all domains utilize." (Zhamak Dehghani, "Data Mesh: Delivering Data-Driven Value at Scale", 2021)
"Data mesh is a solution for organizations that experience scale and complexity, where existing data warehouse or lake solutions have become blockers in their ability to get value from data at scale and across many functions of their business, in a timely fashion and with less friction." (Zhamak Dehghani, "Data Mesh: Delivering Data-Driven Value at Scale", 2021)
"Data Mesh must allow for data models to change continuously without fatal impact to downstream data consumers, or slowing down access to data as a result of synchronizing change of a shared global canonical model. Data Mesh achieves this by localizing change to domains by providing autonomy to domains to model their data based on their most intimate understanding of the business without the need for central coordinations of change to a single shared canonical model." (Zhamak Dehghani, "Data Mesh: Delivering Data-Driven Value at Scale", 2021)
"Data mesh [...] reduces points of centralization that act as coordination bottlenecks. It finds a new way of decomposing the data architecture without slowing the organization down with synchronizations. It removes the gap between where the data originates and where it gets used and removes the accidental complexities - aka pipelines - that happen in between the two planes of data. Data mesh departs from data myths such as a single source of truth, or one tightly controlled canonical data model." (Zhamak Dehghani, "Data Mesh: Delivering Data-Driven Value at Scale", 2021)
"Data mesh relies on a distributed architecture that consists of domains. Each domain is an independent unit of data and its associated storage and compute components. When an organization contains various product units, each with its own data needs, each product team owns a domain that is operated and governed independently by the product team. […] Data mesh has a unique value proposition, not just offering scale of infrastructure and scenarios but also helping shift the organization’s culture around data," (Rukmani Gopalan, "The Cloud Data Lake: A Guide to Building Robust Cloud Data Architecture", 2022)
"Data has historically been treated as a second-class citizen, as a form of exhaust or by-product emitted by business applications. This application-first thinking remains the major source of problems in today’s computing environments, leading to ad hoc data pipelines, cobbled together data access mechanisms, and inconsistent sources of similar-yet-different truths. Data mesh addresses these shortcomings head-on, by fundamentally altering the relationships we have with our data. Instead of a secondary by-product, data, and the access to it, is promoted to a first-class citizen on par with any other business service." (Adam Bellemare,"Building an Event-Driven Data Mesh: Patterns for Designing and Building Event-Driven Architectures", 2023)
"Data mesh architectures are inherently decentralized, and significant responsibility is delegated to the data product owners. A data mesh also benefits from a degree of centralization in the form of data product compatibility and common self-service tooling. Differing opinions, preferences, business requirements, legal constraints, technologies, and technical debt are just a few of the many factors that influence how we work together." (Adam Bellemare, "Building an Event-Driven Data Mesh: Patterns for Designing and Building Event-Driven Architectures", 2023)
"The data mesh is an exciting new methodology for managing data at large. The concept foresees an architecture in which data is highly distributed and a future in which scalability is achieved by federating responsibilities. It puts an emphasis on the human factor and addressing the challenges of managing the increasing complexity of data architectures." (Piethein Strengholt, "Data Management at Scale: Modern Data Architecture with Data Mesh and Data Fabric" 2nd Ed., 2023)
"A data mesh splits the boundaries of the exchange of data into multiple data products. This provides a unique opportunity to partially distribute the responsibility of data security. Each data product team can be made responsible for how their data should be accessed and what privacy policies should be applied." (Aniruddha Deswandikar,"Engineering Data Mesh in Azure Cloud", 2024)
"A data mesh is a decentralized data architecture with four specific characteristics. First, it requires independent teams within designated domains to own their analytical data. Second, in a data mesh, data is treated and served as a product to help the data consumer to discover, trust, and utilize it for whatever purpose they like. Third, it relies on automated infrastructure provisioning. And fourth, it uses governance to ensure that all the independent data products are secure and follow global rules."(James Serra, "Deciphering Data Architectures", 2024)
"At its core, a data fabric is an architectural framework, designed to be employed within one or more domains inside a data mesh. The data mesh, however, is a holistic concept, encompassing technology, strategies, and methodologies." (James Serra, "Deciphering Data Architectures", 2024)
"It is very important to understand that data mesh is a concept, not a technology. It is all about an organizational and cultural shift within companies. The technology used to build a data mesh could follow the modern data warehouse, data fabric, or data lakehouse architecture - or domains could even follow different architectures." (James Serra, "Deciphering Data Architectures", 2024)
"To explain a data mesh in one sentence, a data mesh is a centrally managed network of decentralized data products. The data mesh breaks the central data lake into decentralized islands of data that are owned by the teams that generate the data. The data mesh architecture proposes that data be treated like a product, with each team producing its own data/output using its own choice of tools arranged in an architecture that works for them. This team completely owns the data/output they produce and exposes it for others to consume in a way they deem fit for their data." (Aniruddha Deswandikar,"Engineering Data Mesh in Azure Cloud", 2024)
"With all the hype, you would think building a data mesh is the answer to all of these 'problems' with data warehousing. The truth is that while data warehouse projects do fail, it is rarely because they can’t scale enough to handle big data or because the architecture or the technology isn’t capable. Failure is almost always because of problems with the people and/or the process, or that the organization chose the completely wrong technology." (James Serra, "Deciphering Data Architectures", 2024)
About Me
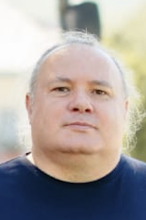
- Adrian
- Koeln, NRW, Germany
- IT Professional with more than 25 years experience in IT in the area of full life-cycle of Web/Desktop/Database Applications Development, Software Engineering, Consultancy, Data Management, Data Quality, Data Migrations, Reporting, ERP implementations & support, Team/Project/IT Management, etc.