A Software Engineer and data professional's blog on SQL, data, databases, data architectures, data management, programming, Software Engineering, Project Management, ERP implementation and other IT related topics.
Pages
- 🏠Home
- 🗃️Posts
- 🗃️Definitions
- 🏭Fabric
- ⚡Power BI
- 🔢SQL Server
- 📚Data
- 📚Engineering
- 📚Management
- 📚SQL Server
- 📚Systems Thinking
- ✂...Quotes
- 🧾D365: GL
- 💸D365: AP
- 💰D365: AR
- 👥D365: HR
- ⛓️D365: SCM
- 🔤Acronyms
- 🪢Experts
- 🗃️Quotes
- 🔠Dataviz
- 🔠D365
- 🔠Fabric
- 🔠Engineering
- 🔠Management
- 🔡Glossary
- 🌐Resources
- 🏺Dataviz
- 🗺️Social
- 📅Events
- ℹ️ About
10 February 2018
🔬Data Science: Data Mining (Definitions)
09 February 2018
🔬Data Science: Normalization (Definitions)
"Mathematical transformations to generate a new set of values that map onto a different range." (Glenn J Myatt, "Making Sense of Data: A Practical Guide to Exploratory Data Analysis and Data Mining", 2006)
[Min–max normalization:] "Normalizing a variable value to a predetermine range." (Glenn J Myatt, "Making Sense of Data: A Practical Guide to Exploratory Data Analysis and Data Mining", 2006)
[function point normalization:] "Dividing a metric by the project’s function points to allow you to compare projects of different sizes and complexities." (Rod Stephens, "Beginning Software Engineering", 2015)
"For metrics, performing some calculation on a metric to account for possible differences in project size or complexity. Two general approaches are size normalization and function point normalization." (Rod Stephens, "Beginning Software Engineering", 2015)
[size normalization:] "For metrics, dividing a metric by an indicator of size such as lines of code or days of work. For example, bugs/KLOC tells you how buggy the code is normalized for the size of the project." (Rod Stephens, "Beginning Software Engineering", 2015)
07 February 2018
🔬Data Science: Hadoop (Definitions)
"An Apache-managed software framework derived from MapReduce and Bigtable. Hadoop allows applications based on MapReduce to run on large clusters of commodity hardware. Hadoop is designed to parallelize data processing across computing nodes to speed computations and hide latency. Two major components of Hadoop exist: a massively scalable distributed file system that can support petabytes of data and a massively scalable MapReduce engine that computes results in batch." (Marcia Kaufman et al, "Big Data For Dummies", 2013)
"An open-source software platform developed by Apache Software Foundation for data-intensive applications where the data are often widely distributed across different hardware systems and geographical locations." (Kenneth A Shaw, "Integrated Management of Processes and Information", 2013)
"Technology designed to house Big Data; a framework for managing data" (Daniel Linstedt & W H Inmon, "Data Architecture: A Primer for the Data Scientist", 2014)
"an Apache-managed software framework derived from MapReduce. Big Table Hadoop enables applications based on MapReduce to run on large clusters of commodity hardware. Hadoop is designed to parallelize data processing across computing nodes to speed up computations and hide latency. The two major components of Hadoop are a massively scalable distributed file system that can support petabytes of data and a massively scalable MapReduce engine that computes results in batch." (Judith S Hurwitz, "Cognitive Computing and Big Data Analytics", 2015)
"An open-source framework that is built to process and store huge amounts of data across a distributed file system." (Jason Williamson, "Getting a Big Data Job For Dummies", 2015)
"Open-source software framework for distributed storage and distributed processing of Big Data on clusters of commodity hardware." (Hamid R Arabnia et al, "Application of Big Data for National Security", 2015)
"A batch processing infrastructure that stores fi les and distributes work across a group of servers. The infrastructure is composed of HDFS and MapReduce components. Hadoop is an open source software platform designed to store and process quantities of data that are too large for just one particular device or server. Hadoop’s strength lies in its ability to scale across thousands of commodity servers that don’t share memory or disk space." (Benoy Antony et al, "Professional Hadoop®", 2016)
"Apache Hadoop is an open-source framework for processing large volume of data in a clustered environment. It uses simple MapReduce programming model for reliable, scalable and distributed computing. The storage and computation both are distributed in this framework." (Kaushik Pal, 2016)
"A framework that allow for the distributed processing for large datasets." (Neha Garg & Kamlesh Sharma, "Machine Learning in Text Analysis", 2020)
"Hadoop is an open source implementation of the MapReduce paper. Initially, Hadoop required that the map, reduce, and any custom format readers be implemented and deployed to the cluster. Eventually, higher level abstractions were developed, like Apache Hive and Apache Pig." (Alex Thomas, "Natural Language Processing with Spark NLP", 2020)
"A batch processing infrastructure that stores files and distributes work across a group of servers." (Oracle)
"an open-source framework that is built to enable the process and storage of big data across a distributed file system." (Analytics Insight)
"Apache Hadoop is an open-source, Java-based software platform that manages data processing and storage for big data applications. Hadoop works by distributing large data sets and analytics jobs across nodes in a computing cluster, breaking them down into smaller workloads that can be run in parallel. Hadoop can process both structured and unstructured data, and scale up reliably from a single server to thousands of machines." (Databricks) [source]
"Hadoop is an open source software framework for storing and processing large volumes of distributed data. It provides a set of instructions that organizes and processes data on many servers rather than from a centralized management nexus." (Informatica) [source]
🔬Data Science: Semantics (Definitions)
"The meaning of a model that is well-formed according to the syntax of a language." (Anneke Kleppe et al, "MDA Explained: The Model Driven Architecture: Practice and Promise", 2003)
"The part of language concerned with meaning. For example, the phrases 'my mother’s brother' and 'my uncle' are two ways of saying the same thing and, therefore, have the same semantic value." (Craig F Smith & H Peter Alesso, "Thinking on the Web: Berners-Lee, Gödel and Turing", 2008)
"The study of meaning (often the meaning of words). In business systems we are concerned with making the meaning of data explicit (structuring unstructured data), as well as making it explicit enough that an agent could reason about it." (Danette McGilvray, "Executing Data Quality Projects", 2008)
"The branch of philosophy concerned with describing meaning." (David C Hay, "Data Model Patterns: A Metadata Map", 2010)
"Having to do with meaning, usually of words and/or symbols (the syntax). Part of semiotic theory." (DAMA International, "The DAMA Dictionary of Data Management", 2011)
"The study of the meaning behind the syntax (signs and symbols) of a language or graphical expression of something. The semantics can only be understood through the syntax. The syntax is like the encoded representation of the semantics." (DAMA International, "The DAMA Dictionary of Data Management", 2011)
"The study of meaning. In the context of Big Data, semantics is the technique of creating meaningful assertions about data objects. A meaningful assertion, as used here, is a triple consisting of an identified data object, a data value, and a descriptor for the data value. In practical terms, semantics involves making assertions about data objects (i.e., making triples), combining assertions about data objects (i.e., merging triples), and assigning data objects to classes; hence relating triples to other triples. As a word of warning, few informaticians would define semantics in these terms, but I would suggest that most definitions for semantics would be functionally equivalent to the definition offered here." (Jules H Berman, "Principles of Big Data: Preparing, Sharing, and Analyzing Complex Information", 2013)
"Set of mappings forming a representation in order to define the meaningful information of the data." (Hamid R Arabnia et al, "Application of Big Data for National Security", 2015)
"Semantics is a branch of linguistics focused on the meaning communicated by language." (Alex Thomas, "Natural Language Processing with Spark NLP", 2020)
06 February 2018
🔬Data Science: Data Profiling (Definitions)
🔬Data Science: Pig (Definitions)
"A programming interface for programmers to create MapReduce jobs within Hadoop." (Jason Williamson, "Getting a Big Data Job For Dummies", 2015)
"A programming language designed to handle any type of data. Pig helps users to focus more on analyzing large datasets and less time writing map programs and reduce programs. Like Hive and Impala, Pig is a high-level platform used for creating MapReduce programs more easily. The programming language Pig uses is called Pig Latin, and it allows you to extract, transform, and load (ETL) data at a very high level. This greatly reduces the effort if this was written in JAVA code; PIG is only a fraction of that." (Benoy Antony et al, "Professional Hadoop®", 2016)
"An open-source platform for analyzing large data sets that consists of the following: (1) Pig Latin scripting language; (2) Pig interpreter that converts Pig Latin scripts into MapReduce jobs. Pig runs as a client application." (Oracle)
05 February 2018
🔬Data Science: Machine Learning [ML] (Definitions)
04 February 2018
🔬Data Science: Artificial Intelligence [AI] (Definitions)
"A computer would deserve to be called intelligent if it could deceive a human into believing that it was human." (Alan Turing, "Computing Machinery and Intelligence", 1950)
"Artificial intelligence is the science of making machines do things that would require intelligence if done by men." (Marvin Minsky, 1968)
"Artificial intelligence comprises methods, tools, and systems for solving problems that normally require the intelligence of humans. The term intelligence is always defined as the ability to learn effectively, to react adaptively, to make proper decisions, to communicate in language or images in a sophisticated way, and to understand." (Nikola K Kasabov, "Foundations of Neural Networks, Fuzzy Systems, and Knowledge Engineering", 1996)
🔬Data Science: Metamodel (Definitions)
"Model of a model that dictates the rules for creation of modeling mechanisms like the UML" (Bhuvan Unhelkar, "Process Quality Assurance for UML-Based Projects", 2002)
"A description or definition of a well-defined language in the form of a model." (Anneke Kleppe et al, "MDA Explained: The Model Driven Architecture™: Practice and Promise", 2003)
"A model that defines other models. The UML metamodel defines the element types of the UML, such as Classifier." (Craig Larman, "Applying UML and Patterns", 2004)
"A description of a model. A meta model refers to the rules that define the structure a model can have. In other words, a meta model defines the formal structure and elements of a model." (Nicolai M Josuttis, "SOA in Practice", 2007)
"The model of a language used to develop systems. In the case of UML, the definition of UML itself is the metamodel." (Bruce P Douglass, "Real-Time Agility: The Harmony/ESW Method for Real-Time and Embedded Systems Development", 2009)
"A description of a model. A meta-model refers to the rules that define the structure a model can have. In other words, a meta-model defines the formal structure and elements of a model." (David Lyle & John G Schmidt, "Lean Integration", 2010)
"1.Generally, a model that specifies one or more other models. 2.In Meta-data Management, a model of a meta-data system or a data model for a meta-data repository." (DAMA International, "The DAMA Dictionary of Data Management", 2011)
"Model that describes how and with what the architecture will be described in a structural way (model of the model)." (Gilbert Raymond & Philippe Desfray, "Modeling Enterprise Architecture with TOGAF", 2014)
"When common sets of design decisions can be identified that are not specific to any one domain, they often become systematized in textbooks and in design practices, and may eventually be designed into standard formats and architectures for creating organizing systems. These formally recognized sets of design decisions are known as abstract models or metamodels. Metamodels describe structures commonly found in resource descriptions and other information resources, regardless of the specific domain." (Robert J Glushko, "The Discipline of Organizing: Professional Edition" 4th Ed., 2016)
02 February 2018
🔬Data Science: Sensitivity Analysis (Definitions)
"The practice of changing a variable in a financial model or forecast to determine how a change in that variable affects the overall outcome. For example, to consider the way in which a change in price might affect the gross profit in a product forecast, one might vary the price in small increments and recompute the figures to see how gross profit changes." (Steven Haines, "The Product Manager's Desk Reference", 2008)
"Sensitivity analysis is a methodology for assessing whether an empirical effect is a valid causal effect. The basic idea is to simulate the change in the empirical effect that would result under plausible assumptions about the possible impact of the most likely sources of bias." (Herbert I Weisberg, "Bias and Causation: Models and Judgment for Valid Comparisons", 2010)
"Use of quantitative and qualitative information to study changes in results that would occur with changes in various assumptions. Also see best-case and worst-case scenario." (Leslie G Eldenburg & Susan K Wolcott, "Cost Management 2nd Ed", 2011)
"Study of the impact that changes in one or more parts of a model have on other parts or the outcome." (Linda Volonino & Efraim Turban, "Information Technology for Management" 8th Ed, 2011)
"A quantitative risk analysis and modeling technique used to help determine which risks have the most potential impact on the project. It examines the extent to which the uncertainty of each project element affects the objective being examined when all other uncertain elements are held at their baseline values. The typical display of results is in the form of a tornado diagram." (Cynthia Stackpole, "PMP® Certification All-in-One For Dummies®", 2011)
"A form of simulation modeling that focuses specifically on identifying the upper and lower bounds of model outputs given a series of inputs with specific variance." (Evan Stubbs, "Delivering Business Analytics: Practical Guidelines for Best Practice", 2013)
"An analysis used in mathematical modelling, where the sensitivity of model results to variations in a particular variable is studied." (K N Krishnaswamy et al, "Management Research Methodology: Integration of Principles, Methods and Techniques", 2016)
"An analysis technique to determine which individual project risks or other sources of uncertainty have the most potential impact on project outcomes, by correlating variations in project outcomes with variations in elements of a quantitative risk analysis model." (Project Management Institute, "A Guide to the Project Management Body of Knowledge (PMBOK® Guide )", 2017)
"An analysis that involves calculating a decision model multiple times with different inputs so a modeler can analyze the alternative results." (Ciara Heavin & Daniel J Power, "Decision Support, Analytics, and Business Intelligence 3rd Ed.", 2017)
"A technique used to determine how different values of an independent variable will impact a particular dependent variable under a given set of assumptions. It allows an analyst to determine whether a statistical finding will remain consistent under a variety of conditions. |" (Jonathan Ferrar et al, "The Power of People: Learn How Successful Organizations Use Workforce Analytics To Improve Business Performance", 2017)
01 February 2018
🔬Data Science: Data Analysis (Definitions)
"Obtaining information from measured or observed data." (Ildiko E Frank & Roberto Todeschini, "The Data Analysis Handbook", 1994)
"Refers to the process of organizing, summarizing and visualizing data in order to draw conclusions and make decisions." (Glenn J Myatt, "Making Sense of Data: A Practical Guide to Exploratory Data Analysis and Data Mining", 2006)
"A combination of human activities and computer processes that answer a research question or confirm a research hypotheses. It answers the question from data files, using empirical methods such as correlation, t-test, content analysis, or Mill’s method of agreement." (Jens Mende, "Data Flow Diagram Use to Plan Empirical Research Projects", 2009)
"The study and presentation of data to create information and knowledge." (DAMA International, "The DAMA Dictionary of Data Management", 2011)
"Process of applying statistical techniques to evaluate data." (Sally-Anne Pitt, "Internal Audit Quality", 2014)
"Research phase in which data gathered from observing participants are analysed, usually with statistical procedures." (K N Krishnaswamy et al, "Management Research Methodology: Integration of Principles, Methods and Techniques", 2016)
🔬Data Science: Exploratory Data Analysis (Definitions)
"Exploratory data analysis (EDA) is a collection of techniques that reveal (or search for) structure in a data set before calculating any probabilistic model. Its purpose is to obtain information about the data distribution (univariate or multivariate), about the presence of outliers and clusters, to disclose relationships and correlations between objects and/or variables." (Ildiko E Frank & Roberto Todeschini, "The Data Analysis Handbook", 1994)
"Processes and methods for exploring patterns and trends in the data that are not known prior to the analysis. It makes heavy use of graphs, tables, and statistics." (Glenn J Myatt, "Making Sense of Data: A Practical Guide to Exploratory Data Analysis and Data Mining", 2007)
"The process of analyzing data to suggest hypotheses using statistical tools, which can then be tested." (DAMA International, "The DAMA Dictionary of Data Management", 2011)
"In statistics, exploratory data analysis is an approach to analyzing datasets to summarize their main characteristics, often with visual methods." (Keith Holdaway, "Harness Oil and Gas Big Data with Analytics", 2014)
"Process in which data patterns guide the analysis or suggest revisions to the preliminary data analysis plan." (K N Krishnaswamy et al, "Management Research Methodology: Integration of Principles, Methods and Techniques", 2016)
"Exploratory Data Analysis is about taking a dataset and extracting the most important information from it, in such a way that it is possible to get an idea of what the data looks like." (Richard M Reese et al, Java: Data Science Made Easy, 2017)
🔬Data Science: MapReduce (Definitions)
"A data processing and aggregation paradigm consisting of a 'map' phase that selects data and a 'reduce' phase that transforms the data. In MongoDB, you can run arbitrary aggregations over data using map-reduce." (MongoDb, "Glossary", 2008)
"A divide-and-conquer strategy for processing large data sets in parallel. In the 'map' phase, the data sets are subdivided. The desired computation is performed on each subset. The 'reduce' phase combines the results of the subset calculations into a final result. MapReduce frameworks handle the details of managing the operations and the nodes they run on, including restarting operations that fail for some reason. The user of the framework only has to write the algorithms for mapping and reducing the data sets and computing with the subsets." (Dean Wampler & Alex Payne, "Programming Scala", 2009)
"A method by which computationally intensive problems can be processed on multiple computers in parallel. The method can be divided into a mapping step and a reducing step. In the mapping step, a master computer divides a problem into smaller problems that are distributed to other computers. In the reducing step, the master computer collects the output from the other computers. Although MapReduce is intended for Big Data resources, holding petabytes of data, most Big Data problems do not require MapReduce." (Jules H Berman, "Principles of Big Data: Preparing, Sharing, and Analyzing Complex Information", 2013)
"An early Big Data (before this term became popular) programming solution originally developed by Google for parallel processing using very large data sets distributed across a number of computing and storage systems. A Hadoop implementation of MapReduce is now available." (Kenneth A Shaw, "Integrated Management of Processes and Information", 2013)
"Designed by Google as a way of efficiently executing a set of functions against a large amount of data in batch mode. The 'map' component distributes the programming problem or tasks across a large number of systems and handles the placement of the tasks in a way that balances the load and manages recovery from failures. After the distributed computation is completed, another function called 'reduce' aggregates all the elements back together to provide a result." (Marcia Kaufman et al, "Big Data For Dummies", 2013)
"A programming model
consisting of two logical steps - Map and Reduce - for processing massively
parallelizable problems across extremely large datasets using a large cluster
of commodity computers." (Haoliang Wang et al, "Accessing Big Data in the Cloud
Using Mobile Devices", Handbook of Research on Cloud Infrastructures for Big
Data Analytics, 2014)
"Algorithm that is used to split massive data sets among many commodity hardware pieces in an effort to reduce computing time." (Billie Anderson & J Michael Hardin, "Harnessing the Power of Big Data Analytics", Encyclopedia of Business Analytics and Optimization, 2014)
"MapReduce is a parallel programming model proposed by Google and is used to distribute computing on clusters of computers for processing large data sets." (Jyotsna T Wassan, "Emergence of NoSQL Platforms for Big Data Needs", Encyclopedia of Business Analytics and Optimization, 2014)
"A concept which is an abstraction of the primitives ‘map’ and ‘reduce’. Most of the computations are carried by applying a ‘map’ operation to each global record in order to generate key/value pairs and then apply the reduce operation in order to combine the derived data appropriately." (P S Shivalkar & B K Tripathy, "Rough Set Based Green Cloud Computing in Emerging Markets", Encyclopedia of Information Science and Technology 3rd Ed., 2015)
"A programming model that uses a divide and conquer method to speed-up processing large datasets, with a special focus on semi-structured data." (Alfredo Cuzzocrea & Mohamed M Gaber, "Data Science and Distributed Intelligence", Encyclopedia of Information Science and Technology 3rd Ed., 2015)
"MapReduce is a programming model for general-purpose
parallelization of data-intensive processing. MapReduce divides the processing
into two phases: a mapping phase, in which data is broken up into chunks that
can be processed by separate threads - potentially running on separate
machines; and a reduce phase, which combines the output from the mappers into
the final result." (Guy Harrison, "Next Generation Databases: NoSQL, NewSQL, and
Big Data", 2015)
"MapReduce is a technological framework for processing parallelize-able problems across huge data sets using a large number of computers (nodes). […] MapReduce consists of two major steps: 'Map' and 'Reduce'. They are similar to the original Fork and Join operations in distributed systems, but they can consider a large number of computers that can be constructed based on the Internet cloud. In the Map-step, the master computer (a node) first divides the input into smaller sub-problems and then distributes them to worker computers (worker nodes). A worker node may also be a sub-master node to distribute the sub-problem into even smaller problems that will form a multi-level structure of a task tree. The worker node can solve the sub-problem and report the results back to its upper level master node. In the Reduce-step, the master node will collect the results from the worker nodes and then combine the answers in an output (solution) of the original problem." (Li M Chen et al, "Mathematical Problems in Data Science: Theoretical and Practical Methods", 2015)
"A programming model which process massive amounts of
unstructured data in parallel and distributed cluster of processors." (Fatma
Mohamed et al, "Data Streams Processing Techniques Data Streams Processing
Techniques", Handbook of Research on Machine Learning Innovations and Trends,
2017)
"A data processing framework of Hadoop which provides data
intensive computation of large data sets by dividing tasks across several
machines and finally combining the result." (Rupali Ahuja, "Hadoop Framework for
Handling Big Data Needs", Handbook of Research on Big Data Storage and
Visualization Techniques, 2018)
"A high-level programming model, which uses the “map” and “reduce” functions, for processing high volumes of data." (Carson K.-S. Leung, "Big Data Analysis and Mining", Encyclopedia of Information Science and Technology 4th Ed., 2018)
"Is a computational paradigm for processing massive datasets in parallel if the computation fits a three-step pattern: map, shard and reduce. The map process is a parallel one. Each process executes on a different part of data and produces (key, value) pairs. The shard process collects the generated pairs, sorts and partitions them. Each partition is assigned to a different reduce process which produces a single result." (Venkat Gudivada et al, "Database Systems for Big Data Storage and Retrieval", Handbook of Research on Big Data Storage and Visualization Techniques, 2018)
"Is a programming
model or algorithm for the processing of data using a parallel programming
implementation and was originally used for academic purposes associated with
parallel programming techniques. (Soraya Sedkaoui, "Understanding Data Analytics
Is Good but Knowing How to Use It Is Better!", Big Data Analytics for
Entrepreneurial Success, 2019)
"MapReduce is a style of programming based on functional programming that was the basis of Hadoop." (Alex Thomas, "Natural Language Processing with Spark NLP", 2020)
"Is a specific programming model, which as such represents a new approach to solving the problem of processing large amounts of differently structured data. It consists of two functions - Map (sorting and filtering data) and Reduce (summarizing intermediate results), and it is executed in parallel and distributed." (Savo Stupar et al, "Importance of Applying Big Data Concept in Marketing Decision Making", Handbook of Research on Applied AI for International Business and Marketing Applications, 2021)
"A software framework for processing vast amounts of data." (Analytics Insight)
29 January 2018
🔬Data Science: Data Products (Definitions)
"Broadly defined, data means events that are captured and made available for analysis. A data source is a consistent record of these events. And a data product translates this record of events into something that can easily be understood." (Richard Galentino; et al, "Data Fluency: Empowering Your Organization with Effective Data Communication", 2014)
"Self-adapting, broadly applicable economic engines that derive their value from data and generate more data by influencing human behavior or by making inferences or predictions upon new data." (Benjamin Bengfort & Jenny Kim, "Data Analytics with Hadoop", 2016)
"Data products are software applications that derive value from data and in turn generate new data." (Rebecca Bilbro et al, "Applied Text Analysis with Python", 2018)
"[...] a product that facilitates an end goal through the use of data." (Ulrika Jägare, "Data Science Strategy For Dummies", 2019)
"Any computer software that uses data as inputs, produces outputs, and provides feedback based on the output to control the environment is referred to as a data product. A data product is generally based on a model developed during data analysis, for example, a recommendation model that inputs user purchase history and recommends a related item that the user is highly likely to buy." (Suresh K Mukhiya; Usman Ahmed, Hands-On Exploratory Data Analysis with Python, 2020)
"A data product is a product or service whose value is derived from using algorithmic methods on data, and which in turn produces data to be used in the same product, or tangential data products." (Statistics.com)
"A data product, in general terms, is any tool or application that processes data and generates results. […] Data products have one primary objective: to manage, organize and make sense of the vast amount of data that organizations collect and generate. It’s the users’ job to put the insights to use that they gain from these data products, take actions and make better decisions based on these insights." (Sisense) [source]
"A strategy for monetizing an organization’s data by offering it as a product to other parties." (Izenda)
"An information product that is derived from observational data through any kind of computation or processing. This includes aggregation, analysis, modelling, or visualization processes." (Fixed-Point Open Ocean Observatories)
"Data set or data set series that conforms to a data product specification." (ISO 19131)
28 January 2018
🔬Data Science: Regularization (Definitions)
"It is a formal concept based on fuzzy topology that removes
geometric anomalies on fuzzy regions." (Markus Schneider, "Fuzzy Spatial Data
Types for Spatial Uncertainty Management in Databases", 2008)
"It is any method of preventing overfitting of data by a model and it is used for solving ill-conditioned parameter-estimation problems." (Cecilio Angulo & Luis Gonzalez-Abril, "Support Vector Machines", 2009)
"Optimization of both complexity and performance of a neural
network following a linear aggregation or a multi-objective algorithm." (M P
Cuéllar et al, "Multi-Objective Training of Neural Networks", 2009)
"Including a term in the error function such that the training process favours networks of moderate size and complexity, that is, networks with small weights and few hidden units. The goal is to avoid overfitting and support generalization." (Frank Padberg, "Counting the Hidden Defects in Software Documents", 2010)
"It refers to the procedure of bringing in additional
knowledge to solve an ill-posed problem or to avoid overfitting. This
information appears habitually as a penalty term for complexity, such as
constraints for smoothness or bounds on the norm." (Vania V Estrela et al, "Total
Variation Applications in Computer Vision", 2016)
"This is a general method to avoid overfitting by applying additional constraints to the model that is learned. A common approach is to make sure the model weights are, on average, small in magnitude." (Rayid Ghani & Malte Schierholz, "Machine Learning", 2017)
"Regularization is a method of penalizing complex models to reduce their variance. Specifically, a penalty term is added to the loss function we are trying to minimize [...]" (Chris Albon, "Machine Learning with Python Cookbook", 2018)
"Regularization, generally speaking, is a wide range of ML techniques aimed at reducing overfitting of the models while maintaining theoretical expressive power." (Jonas Teuwen & Nikita Moriakov, "Convolutional neural networks", 2020)
About Me
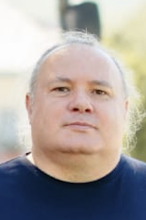
- Adrian
- Koeln, NRW, Germany
- IT Professional with more than 24 years experience in IT in the area of full life-cycle of Web/Desktop/Database Applications Development, Software Engineering, Consultancy, Data Management, Data Quality, Data Migrations, Reporting, ERP implementations & support, Team/Project/IT Management, etc.